학술논문
머신러닝 기법을 활용한 중학생의 영어과목 학업성취 예측 및 주요 요인 탐색
이용수 0
- 영문명
- Predicting Middle School Students' English Academic Achievement and Exploring Key Factors Using Machine Learning Techniques
- 발행기관
- 학습자중심교과교육학회
- 저자명
- 황수진(Sujin Hwang) 김현철(Hyunchul Kim)
- 간행물 정보
- 『학습자중심교과교육연구』제25권 7호, 638~655쪽, 전체 18쪽
- 주제분류
- 사회과학 > 교육학
- 파일형태
- 발행일자
- 2025.04.15
4,960원
구매일시로부터 72시간 이내에 다운로드 가능합니다.
이 학술논문 정보는 (주)교보문고와 각 발행기관 사이에 저작물 이용 계약이 체결된 것으로, 교보문고를 통해 제공되고 있습니다.
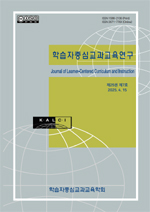
국문 초록
목적 본 연구에서는 다양한 머신러닝 기법들로 중학생의 영어교과 학업성취를 예측하고, 주요 예측요인들을 상대적 중요도를 탐색하여 학년별로 비교·분석하였다.
방법 이를 위하여 최근접이웃기법(KNN), 의사결정나무(C5.0), 랜덤포레스트(RF), 서포트벡터머신(SVM), 신경망(NNET) 등의 머신러닝 기법을 활용하여, 학년별로 기법별 예측력을 비교 및 분석하고, 주요한 예측요인들을 파악하고자 하였다. 학업성취를 학년별로 비교하기 위하여 영어과목 수직척도점수를 활용하였고, 학업성취를 예측하기 위한 요인은 개인수준, 가정수준, 그리고 학교수준으로 나누어 살펴보았다. 이를 위하여 경기교육종단연구(GEPS) 중학교 자료를 사용하였고, 데이터정제를 통해 중학교 1학년 499명, 중학교 2학년 486명, 그리고 중학교 3학년 457명을 분석에 투입하였다. 또한 중학생의 학업성취 예측을 위하여 학생수준 394개, 가정수준 45개, 학교수준 106개의 요인을 투입하여 결과를 비교 및 분석 하였다.
결과 연구 결과를 살펴보면 중학교 1·2학년에서는 서포트벡터머신(SVM), 3학년에서는 의사결정나무(C5.0)이 가장 높은 예측 성능을 보였다. 또한, 학업 성취의 주요 예측 요인을 분석한 결과, 모든 학년에서 ‘직전 학년 영어 성적’, ‘영어 수업 이해도’, ‘영어 교과 효능감’, ‘학습 태도’가 중요한 영향 요인으로 확인되었다. 반면, 부모 학력이나 가구 소득과 같은 가정 환경 요인의 영향력은 상대적으로 낮은 경향을 보였다. 특히, 학년이 올라갈수록 학교 환경 요인(학교 만족도, 학급 분위기 등)의 중요성이 점진적으로 증가하는 패턴이 나타났다.
결론 본 연구에서는 여러 머신러닝 기법을 활용하여 중학교 학생의 학년별 영어 과목 학업 성취를 예측하고, 주요 예측 요인을 비교·분석하였다. 또한, 다양한 머신러닝 기법을 비교하여 학년별 최적의 예측 모델을 확인하고, 학업 성취의 주요 영향 요인을 도출하였다. 이러한 연구 결과는 향후 수준별 맞춤형 학습 지원의 기초 자료로 활용될 수 있으며, 개별화된 학습 지원 및 교육정책 수립에 기여할 것으로 기대된다.
영문 초록
Objectives This study aims to predict middle school students' academic achievement in English using various machine learning techniques and to explore the relative importance of key predictive factors by comparing and analyzing them across different grade levels.
Methods To achieve this, machine learning techniques such as k-Nearest Neighbors (KNN), Decision Tree (C5.0), Random Forest (RF), Support Vector Machine (SVM), and Neural Network (NNET) were utilized. The predictive accuracy of each model was compared and analyzed for each grade level, and the key predictive factors were identified. To compare academic achievement across grade levels, vertical scale scores for each subject were used. The predictive factors were categorized into three levels: individual, family, and school. For data collection, this study used middle school data from the Gyeonggi Education Panel Study (GEPS). After data preprocessing, a total of 499 first-year, 486 second-year, and 457 third-year middle school students were included in the analysis. In total, 394 individual-level variables, 45 family-level variables, and 106 school-level variables were used to predict students' academic achievement, and the results were compared and analyzed accordingly.
Results The findings indicate that Support Vector Machine (SVM) achieved the highest predictive performance for first- and second-year students, while Decision Tree (C5.0) performed best for third-year students. The analysis of key predictive factors revealed that previous-year English grades, English class comprehension, English self-efficacy, and learning attitude were consistently significant predictors across all grade levels. On the other hand, family-related factors such as parental education level and household income had relatively lower predictive power. Additionally, as grade levels increased, the importance of school environment factors (e.g., school satisfaction, classroom atmosphere) showed a gradual increase.
Conclusions This study utilized various machine learning techniques to predict English academic achievement among middle school students and to compare key predictive factors across different grade levels. Furthermore, the study identified the most suitable predictive model for each grade level by comparing different machine learning algorithms. These findings provide valuable insights for developing customized learning support systems and can serve as foundational data for personalized education strategies and future education policy development.
목차
Ⅰ. 서론
Ⅱ. 연구 방법
Ⅲ. 연구결과
Ⅳ. 결론 및 제언
참고문헌
키워드
해당간행물 수록 논문
- 학습자중심교과교육연구 제25권 7호 목차
- 상호문화적 관점의 한국어 문화교육과정 개발을 위한 기초연구: 이주배경 중등학습자의 요구조사를 중심으로
- 자기주도 환경교육이 대안학교 아동의 기후변화 인식, 지식, 태도와 기후변화 대응 실천역량에 미치는 영향
- 아동과 모의 삶의 만족도 궤적을 활용한 유형화 분석 및 집단별 특성에 관한 연구
- 학습심리적 특성과 디지털 리터러시 교육활동이 디지털 리터러시를 매개로 학생핵심역량에 미치는 효과: 경기도학교교육실태조사 자료를 활용한 분석
- 다문화가정 아동이 지각하는 가족지지가 학교적응에 미치는 영향: 자아존중감과 성취동기의 이중매개효과
- 유아교사의 다문화수용성이 다문화교육역량에 미치는 영향: 인권의식의 매개효과를 중심으로
- 청소년의 정치성향이 정치적 태도에 미치는 영향에 관한 연구
- 간호대학생의 생활스트레스, 대인관계능력, 자기통제력이 스마트폰 중독경향성에 미치는 영향
- 영아 자녀를 둔 어머니의 후속출산의도에 영향을 미치는 변인 분석: 0세아전용 어린이집 이용 어머니를 대상으로
- 초등학생을 위한 협동학습 기반 피지컬 컴퓨팅을 활용한 인공지능 융합 관계 인성 프로젝트 수업이 인공지능 역량과 협력적 문제해결력에 미치는 영향
- 고등학생의 학업성취도 수준별 자아탄력성 및 의사소통능력 차이 분석
- 혁신교수법으로 적용된 방탈출 관련 연구의 서지정보 분석
- 유아교사의 교수창의성 및 수업역량과 유아의 창의성 간의 구조적 관계 분석
- 반려인의 사회적 지지가 주관적 안녕감에 미치는 영향: 반려동물 상호작용의 매개효과를 중심으로
- 고등학교 축구팀 내 소통이 전략적 의사 결정과 경기 운영에 미치는 영향
- 간호학과와 의학과 학부생의 전문직 간 교육(Interprofessional education, IPE)과 가상현실 학습에 대한 인식과 교육요구도
- 간호대학생의 메타인지, 교수-학생 상호작용 및 임상실습교육환경이 전공만족도에 미치는 영향
- 내현적 자기애가 대인관계 유능성에 미치는 영향: 사회적 지지와 자기 자비의 매개 효과
- 중학교 학교자율시간 운영을 위한 교과 융합 선택 과목 교육과정 개발 모형
- 청소년의 자아존중감 향상을 위한 현실치료 집단상담 프로그램 효과에 대한 메타분석
- 방송 뉴스 앵커 K의 ‘분노하기’ 개인 평가어 연구: 체계기능언어학의 평가어 이론의 관점에서
- 교사의 경계선 지능 학생 교육 및 지도 경험과 지원 요구에 관한 현상학적 연구
- 대학생이 지각한 부모의 과보호 양육방식이 내현적 자기애에 미치는 영향: 자기대상욕구의 매개효과
- 국내 디지털 시민성 관련 연구 동향 분석
- 교수-학습 상황에서 사용되는 공감적 의사소통 전략에 대한 다중 사례연구
- 외국인 유학생의 언어능력, 차별 경험, 사회적 지지가 지각된 한국 사회 다문화 수용성에 미치는 영향
- 보험설계사의 교정적 피드백과 조직몰입의 관계: 긍정적 초점변경으로 조절된 개인적 성취감 감소의 매개 효과
- 영유아교사의 세대별 일과 삶의 균형 및 조직 내 인간관계 차이 분석
- 외국인 유학생의 전공선택 동기가 대학생활적응 및 전공만족도와 진로결정에 미치는 영향
- 지역사회 협력학습의 효과와 참여 대학생의 만족도
- 국가장학금 의존도에 영향을 미치는 요인: 사립대학 재정을 중심으로
- 유아교사의 완벽주의 성향과 테크노스트레스가 디지털 역량에 미치는 영향
- 장애위험 영유아의 특징, 조기발견 및 조기개입, 지원에 관한 예비유아교사들의 인식
- 고등학생의 복합외상경험이 사이버비행에 미치는 영향: 우울과 공격성의 매개효과
- 중국 가오카오 수험생이 지각한 스트레스 수준이 학업성과에 미치는 영향 연구: 학업적 자기효능감 및 긍정심리자본의 매개효과를 중심으로
- 일본어 모어 화자의 한글 자형 인식 양상 연구: 비학습자를 중심으로
- 키워드 네트워크와 토픽모델링을 활용한 디지털교과서에 대한 언론보도 분석
- 빅데이터에 기초한 학습공동체에 대한 키워드 네트워크 분석
- 머신러닝 기법을 활용한 중학생의 영어과목 학업성취 예측 및 주요 요인 탐색
- 간호대학생의 전공만족도와 자기효능감이 간호사 이미지에 미치는 영향: 충청북도 소재 대학을 중심으로
- 병렬 말뭉치 기반 L1(한국어) 화자를 위한 L2(한국수어) 교육 연어 탐색 방법 연구
- 자녀가 없는 기혼 초심 청소년상담사의 부모상담 역전이 경험에 관한 현상학적 연구
- 청소년기관 종사자의 이직의도에 영향을 미치는 요인: 직무스트레스, 심리적소진, 긍정심리자본의 구조적 관계분석
- 영유아 관련 교육적 기록화에 관한 연구 동향 분석
- 첨단 과학기술에 대한 중학생의 생각과 그 이유 탐색: 인공지능, 로봇의 특징을 중심으로
- 특수교육 기본교육과정 변천에 따른 중학교 음악 교과 제재곡 분석
- The Pedagogical Effectiveness of Using Plurilingualism and humor for French Students Learning Korean
- 유보통합의 필요성에 관한 보육교사의 인식 연구
- 영유아 학부모의 통합돌봄에 대한 인식 및 요구도 조사: 광주광역시를 중심으로
- 예비 초등영어 교사의 디지털 리터러시 역량 수준 탐색 연구
참고문헌
교보eBook 첫 방문을 환영 합니다!
신규가입 혜택 지급이 완료 되었습니다.
바로 사용 가능한 교보e캐시 1,000원 (유효기간 7일)
지금 바로 교보eBook의 다양한 콘텐츠를 이용해 보세요!
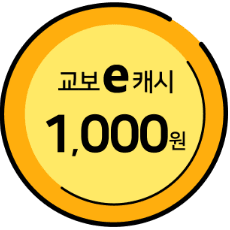