Machine Learning Infrastructure and Best Practices for Software Engineers
2024년 01월 31일 출간
- eBook 상품 정보
- 파일 정보 PDF (12.42MB)
- ISBN 9781837634064
- 지원기기 교보eBook App, PC e서재, 리더기, 웹뷰어
-
교보eBook App
듣기(TTS) 가능
TTS 란?텍스트를 음성으로 읽어주는 기술입니다.
- 전자책의 편집 상태에 따라 본문의 흐름과 다르게 텍스트를 읽을 수 있습니다.
- 이미지 형태로 제작된 전자책 (예 : ZIP 파일)은 TTS 기능을 지원하지 않습니다.
PDF 필기가능 (Android, iOS)
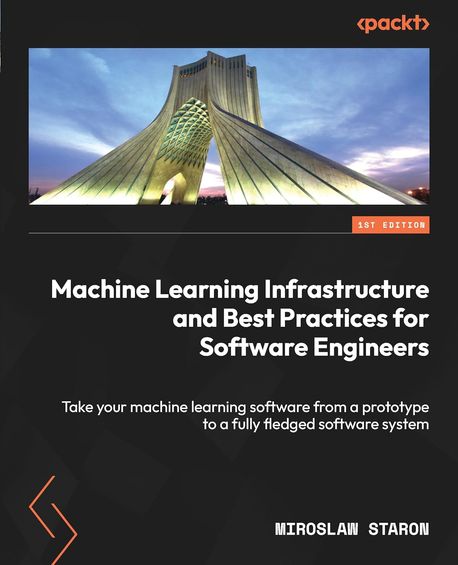
쿠폰적용가 22,500원
10% 할인 | 5%P 적립이 상품은 배송되지 않는 디지털 상품이며,
교보eBook앱이나 웹뷰어에서 바로 이용가능합니다.
카드&결제 혜택
- 5만원 이상 구매 시 추가 2,000P
- 3만원 이상 구매 시, 등급별 2~4% 추가 최대 416P
- 리뷰 작성 시, e교환권 추가 최대 200원
작품소개
이 상품이 속한 분야
▶ Book Description
Although creating a machine learning pipeline or developing a working prototype of a software system from that pipeline is easy and straightforward nowadays, the journey toward a professional software system is still extensive. This book will help you get to grips with various best practices and recipes that will help software engineers transform prototype pipelines into complete software products.
The book begins by introducing the main concepts of professional software systems that leverage machine learning at their core. As you progress, you’ll explore the differences between traditional, non-ML software, and machine learning software. The initial best practices will guide you in determining the type of software you need for your product. Subsequently, you will delve into algorithms, covering their selection, development, and testing before exploring the intricacies of the infrastructure for machine learning systems by defining best practices for identifying the right data source and ensuring its quality.
Towards the end, you’ll address the most challenging aspect of large-scale machine learning systems – ethics. By exploring and defining best practices for assessing ethical risks and strategies for mitigation, you will conclude the book where it all began – large-scale machine learning software.
▶ What You Will Learn
⦁ Identify what the machine learning software best suits your needs
⦁ Work with scalable machine learning pipelines
⦁ Scale up pipelines from prototypes to fully fledged software
⦁ Choose suitable data sources and processing methods for your product
⦁ Differentiate raw data from complex processing, noting their advantages
⦁ Track and mitigate important ethical risks in machine learning software
⦁ Work with testing and validation for machine learning systems
1. Machine Learning Compared to Traditional Software
2. Elements of a Machine Learning Software System
3. Data in Software Systems – Text, Images, Code, Features
4. Data Acquisition, Data Quality and Noise
5. Quantifying and Improving Data Properties
6. Types of Data in ML Systems
7. Feature Engineering for Numerical and Image Data
8. Feature Engineering for Natural Language Data
9. Types of Machine Learning Systems – Feature-Based and Raw Data Based (Deep Learning)
10. Training and evaluation of classical ML systems and neural networks
11. Training and evaluation of advanced algorithms – deep learning, autoencoders, GPT-3
12. Designing machine learning pipelines (MLOps) and their testing
13. Designing and implementation of large scale, robust ML software – a comprehensive example
14. Ethics in data acquisition and management(N.B. Please use the Look Inside option to see further chapters)
▶ What this book covers
⦁ Chapter 1, Machine Learning Compared to Traditional Software, explores where these two types of software systems are most appropriate. We learn about the software development processes that programmers use to create both types of software and we also learn about the classical four types of machine learning software – rule-based, supervised, unsupervised, and reinforcement learning. Finally, we also learn about the different roles of data in traditional and machine learning software.
⦁ Chapter 2, Elements of a Machine Learning System, reviews each element of a professional machine learning system. We start by understanding which elements are important and why. Then, we explore how to create such elements and how to work by putting them together into a single machine learning system – the so-called machine learning pipeline.
⦁ Chapter 3, Data in Software Systems – Text, Images, Code, and Features, introduces three data types – images, texts, and formatted text (program source code). We explore how each of these types of data can be used in machine learning, how they should be annotated, and for what purpose. Introducing these three types of data provides us with the possibility to explore different ways of annotating these sources of data.
⦁ Chapter 4, Data Acquisition, Data Quality, and Noise, dives deeper into topics related to data quality. We go through a theoretical model for assessing data quality and we provide methods and tools to operationalize it. We also look into the concept of noise in machine learning and how to reduce it by using different tokenization methods.
⦁ Chapter 5, Quantifying and Improving Data Properties, dives deeper into the properties of data and how to improve them. In contrast to the previous chapter, we work on feature vectors rather than raw data. The feature vectors are already a transformation of the data; therefore, we can change such properties as noise or even change how the data is perceived. We focus on the processing of text, which is an important part of many machine learning algorithms nowadays. We start by understanding how to transform data into feature vectors using simple algorithms, such as bag of words, so that we can work on feature vectors.
⦁ Chapter 6, Processing Data in Machine Learning Systems, dives deeper into the ways in which data and algorithms are entangled. We talk a lot about data in generic terms, but in this chapter, we explain what kind of data is needed in machine learning systems. We explain the fact that all kinds of data are used in numerical form – either as a feature vector or as more complex feature matrices. Then, we will explain the need to transform unstructured data (e.g., text) into structured data. This chapter will lay the foundations for going deeper into each type of data, which is the content of the next few chapters.
⦁ Chapter 7, Feature Engineering for Numerical and Image Data, focuses on the feature engineering process for numerical and image data. We start by going through the typical methods such as Principal Component Analysis (PCA), which we used previously for visualization. We then move on to more advanced methods such as the t-Student Distribution Stochastic Network Embeddings (t-SNE) and Independent Component Analysis (ICA). What we end up with is the use of autoencoders as a dimensionality reduction technique for both numerical and image data.
⦁ Chapter 8, Feature Engineering for Natural Language Data, explores the first steps that made the transformer (GPT) technologies so powerful – feature extraction from natural language data. Natural language is a special kind of data source in software engineering. With the introduction of GitHub Copilot and ChatGPT, it became evident that machine learning and artificial intelligence tools for software engineering tasks are no longer science fiction.
⦁ Chapter 9, Types of Machine Learning Systems – Feature-Based and Raw Data-Based (Deep Learning), explores different types of machine learning systems. We start from classical machine learning models such as random forest and we move on to convolutional and GPT models, which are called deep learning models. Their name comes from the fact that they use raw data as input and the first layers of the models include feature extraction layers. They are also designed to progressively learn more abstract features as the input data moves through these models. This chapter demonstrates each of these types of models and progresses from classical machine learning to the generative AI models.
⦁ Chapter 10, Training and Evaluation of Classical ML Systems and Neural Networks, goes a bit deeper into the process of training and evaluation. We start with the basic theory behind different algorithms and then we show how they are trained. We start with the classical machine learning models, exemplified by the decision trees. Then, we gradually move toward deep learning where we explore both the dense neural networks and some more advanced types of networks.
⦁ Chapter 11, Training and Evaluation of Advanced ML Algorithms – GPT and Autoencoders, explores how generative AI models work based on GPT and Bidirectional Encoder Representation Transformers (BERT). These models are designed to generate new data based on the patterns that they were trained on. We also look at the concept of autoencoders, where we train an autoencoder to generate new images based on the previously trained data.
⦁ Chapter 12, Designing Machine Learning Pipelines and their Testing, describes how the main goal of MLOps is to bridge the gap between data science and operations teams, fostering collaboration and ensuring that machine learning projects can be effectively and reliably deployed at scale. MLOps helps to automate and optimize the entire machine learning life cycle, from model development to deployment and maintenance, thus improving the efficiency and effectiveness of ML systems in production. In this chapter, we learn how machine learning systems are designed and operated in practice. The chapter shows how pipelines are turned into a software system, with a focus on testing ML pipelines and their deployment at Hugging Face.
⦁ Chapter 13, Designing and Implementation of Large-Scale, Robust ML Software, explains how to integrate the machine learning model with a graphical user interface programmed in Gradio and storage in a database. We use two examples of machine learning pipelines – an example of the model for predicting defects from our previous chapters and a generative AI model to create pictures from a natural language prompt.
⦁ Chapter 14, Ethics in Data Acquisition and Management, starts by exploring a few examples of unethical systems that show bias, such as credit ranking systems that penalize certain minorities. We also explain the problems with using open source data and revealing the identities of subjects. The core of the chapter, however, is the explanation and discussion on ethical frameworks for data management and software systems, including the IEEE and ACM codes of conduct.
⦁ Chapter 15, Ethics in Machine Learning Systems, focuses on the bias in machine learning systems. We start by exploring sources of bias and briefly discussing these sources. We then explore ways to spot biases, how to minimize them, and finally, how to communicate potential biases to the users of our system.
⦁ Chapter 16, Integration of ML Systems in Ecosystems, explains how packaging the ML systems into web services allows us to integrate them into workflows in a very flexible way. Instead of compiling or using dynamically linked libraries, we can deploy machine learning components that communicate over HTTP protocols using JSON protocols. In fact, we have already seen how to use that protocol by using the GPT-3 model that is hosted by OpenAI. In this chapter, we explore the possibility of creating our own Docker container with a pre-trained machine learning model, deploying it, and integrating it with other components.
⦁ Chapter 17, Summary and Where to Go Next, revisits all the best practices and summarizes them per chapter. In addition, we also look into what the future of machine learning and AI may bring to software engineering.
▶ Preface
Machine learning has gained a lot of popularity in recent years. The introduction of large language models such as GPT-3 and 4 only increased the speed of the development of this field. These large language models have become so powerful that it is almost impossible to train them on a local computer. However, this is not necessary at all. These language models provide the ability to create new tools without the need to train them because they can be steered by the context window and the prompt.
In this book, my goal is to show how machine learning models can be trained, evaluated, and tested – both in the context of a small prototype and in the context of a fully-fledged software product. The primary objective of this book is to bridge the gap between theoretical knowledge and practical implementation of machine learning in software engineering. It aims to equip you with the skills necessary to not only understand but also effectively implement and innovate with AI and machine learning technologies in your professional pursuits.
The journey of integrating machine learning into software engineering is as thrilling as it is challenging. As we delve into the intricacies of machine learning infrastructure, this book serves as a comprehensive guide, navigating through the complexities and best practices that are pivotal for software engineers. It is designed to bridge the gap between the theoretical aspects of machine learning and the practical challenges faced during implementation in real-world scenarios.
We begin by exploring the fundamental concepts of machine learning, providing a solid foundation for those new to the field. As we progress, the focus shifts to the infrastructure – the backbone of any successful machine learning project. From data collection and processing to model training and deployment, each step is crucial and requires careful consideration and planning.
A significant portion of the book is dedicated to best practices. These practices are not just theoretical guidelines but are derived from real-life experiences and case studies that my research team discovered during our work in this field. These best practices offer invaluable insights into handling common pitfalls and ensuring the scalability, reliability, and efficiency of machine learning systems.
Furthermore, we delve into the ethics of data and machine learning algorithms. We explore the theories behind ethics in machine learning, look closer into the licensing of data and models, and finally, explore the practical frameworks that can quantify bias in data and models in machine learning.
This book is not just a technical guide; it is a journey through the evolving landscape of machine learning in software engineering. Whether you are a novice eager to learn, or a seasoned professional seeking to enhance your skills, this book aims to be a valuable resource, providing clarity and direction in the exciting and ever-changing world of machine learning.
작가정보
저자(글) Miroslaw Staron
Miroslaw Staron is a professor of Applied IT at the University of Gothenburg in Sweden with a focus on empirical software engineering, measurement, and machine learning. He is currently editor-in-chief of Information and Software Technology and co-editor of the regular Practitioner’s Digest column of IEEE Software. He has authored books on automotive software architectures, software measurement, and action research. He also leads several projects in AI for software engineering and leads an AI and digitalization theme at Software Center. He has written over 200 journal and conference articles.
이 상품의 총서
Klover리뷰 (0)
- - e교환권은 적립일로부터 180일 동안 사용 가능합니다.
- - 리워드는 5,000원 이상 eBook, 오디오북, 동영상에 한해 다운로드 완료 후 리뷰 작성 시 익일 제공됩니다. (2024년 9월 30일부터 적용)
- - 리워드는 한 상품에 최초 1회만 제공됩니다.
- - sam 이용권 구매 상품 / 선물받은 eBook은 리워드 대상에서 제외됩니다.
- 도서나 타인에 대해 근거 없이 비방을 하거나 타인의 명예를 훼손할 수 있는 리뷰
- 도서와 무관한 내용의 리뷰
- 인신공격이나 욕설, 비속어, 혐오 발언이 개재된 리뷰
- 의성어나 의태어 등 내용의 의미가 없는 리뷰
구매 후 리뷰 작성 시, e교환권 100원 적립
문장수집
- 구매 후 90일 이내에 문장 수집 등록 시 e교환권 100원을 적립해 드립니다.
- e교환권은 적립일로부터 180일 동안 사용 가능합니다.
- 리워드는 5,000원 이상 eBook에 한해 다운로드 완료 후 문장수집 등록 시 제공됩니다. (2024년 9월 30일부터 적용)
- 리워드는 한 상품에 최초 1회만 제공됩니다.
- sam 이용권 구매 상품 / 선물받은 eBook / 오디오북·동영상 상품/주문취소/환불 시 리워드 대상에서 제외됩니다.
구매 후 문장수집 작성 시, e교환권 100원 적립
신규가입 혜택 지급이 완료 되었습니다.
바로 사용 가능한 교보e캐시 1,000원 (유효기간 7일)
지금 바로 교보eBook의 다양한 콘텐츠를 이용해 보세요!
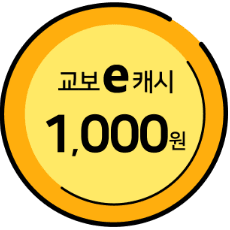
- 구매 후 90일 이내 작성 시, e교환권 100원 (최초1회)
- 리워드 제외 상품 : 마이 > 라이브러리 > Klover리뷰 > 리워드 안내 참고
- 콘텐츠 다운로드 또는 바로보기 완료 후 리뷰 작성 시 익일 제공
가장 와 닿는 하나의 키워드를 선택해주세요.
총 5MB 이하로 jpg,jpeg,png 파일만 업로드 가능합니다.
신고 사유를 선택해주세요.
신고 내용은 이용약관 및 정책에 의해 처리됩니다.
허위 신고일 경우, 신고자의 서비스 활동이 제한될 수
있으니 유의하시어 신중하게 신고해주세요.
이 글을 작성한 작성자의 모든 글은 블라인드 처리 됩니다.
구매 후 90일 이내 작성 시, e교환권 100원 적립
eBook 문장수집은 웹에서 직접 타이핑 가능하나, 모바일 앱에서 도서를 열람하여 문장을 드래그하시면 직접 타이핑 하실 필요 없이 보다 편하게 남길 수 있습니다.
차감하실 sam이용권을 선택하세요.
차감하실 sam이용권을 선택하세요.
선물하실 sam이용권을 선택하세요.
-
보유 권수 / 선물할 권수0권 / 1권
-
받는사람 이름받는사람 휴대전화
- 구매한 이용권의 대한 잔여권수를 선물할 수 있습니다.
- 열람권은 1인당 1권씩 선물 가능합니다.
- 선물한 열람권이 ‘미등록’ 상태일 경우에만 ‘열람권 선물내역’화면에서 선물취소 가능합니다.
- 선물한 열람권의 등록유효기간은 14일 입니다.
(상대방이 기한내에 등록하지 않을 경우 소멸됩니다.) - 무제한 이용권일 경우 열람권 선물이 불가합니다.
첫 구매 시 교보e캐시 지급해 드립니다.
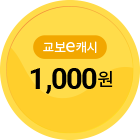
- 첫 구매 후 3일 이내 다운로드 시 익일 자동 지급
- 한 ID당 최초 1회 지급 / sam 이용권 제외
- 구글바이액션을 통해 교보eBook 구매 이력이 없는 회원 대상
- 교보e캐시 1,000원 지급 (유효기간 지급일로부터 7일)