Hands-On Gradient Boosting with XGBoost and scikit-learn
2020년 10월 16일 출간
- eBook 상품 정보
- 파일 정보 PDF (4.80MB)
- ISBN 9781839213809
- 지원기기 교보eBook App, PC e서재, 리더기, 웹뷰어
-
교보eBook App
듣기(TTS) 가능
TTS 란?텍스트를 음성으로 읽어주는 기술입니다.
- 전자책의 편집 상태에 따라 본문의 흐름과 다르게 텍스트를 읽을 수 있습니다.
- 이미지 형태로 제작된 전자책 (예 : ZIP 파일)은 TTS 기능을 지원하지 않습니다.
PDF 필기가능 (Android, iOS)
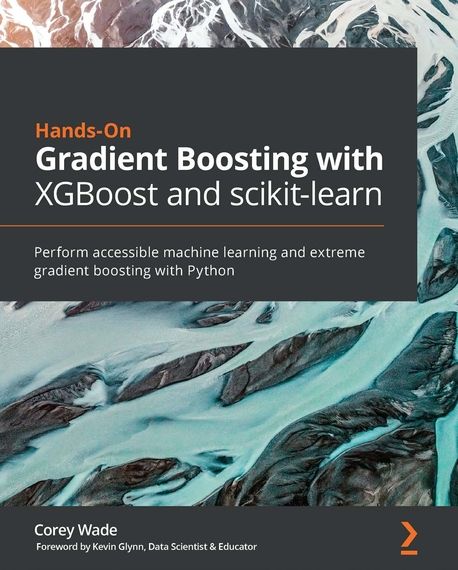
쿠폰적용가 19,800원
10% 할인 | 5%P 적립이 상품은 배송되지 않는 디지털 상품이며,
교보eBook앱이나 웹뷰어에서 바로 이용가능합니다.
카드&결제 혜택
- 5만원 이상 구매 시 추가 2,000P
- 3만원 이상 구매 시, 등급별 2~4% 추가 최대 416P
- 리뷰 작성 시, e교환권 추가 최대 200원
작품소개
이 상품이 속한 분야
▶What You Will Learn
-Build gradient boosting models from scratch
-Develop XGBoost regressors and classifiers with accuracy and speed
-Analyze variance and bias in terms of fine-tuning XGBoost hyperparameters
-Automatically correct missing values and scale imbalanced data
-Apply alternative base learners like dart, linear models, and XGBoost random forests
-Customize transformers and pipelines to deploy XGBoost models
-Build non-correlated ensembles and stack XGBoost models to increase accuracy
▶Key Features
-Get up and running with machine learning and understand how to boost models with XGBoost in no time
-Build real-world machine learning pipelines and fine-tune hyperparameters to achieve optimal results
-Discover tips and tricks and gain innovative insights from XGBoost Kaggle winners
▶Who This Book Is For
This book is for data science professionals and enthusiasts, data analysts, and developers who want to build fast and accurate machine learning models that scale with big data. Proficiency in Python, along with a basic understanding of linear algebra, will help you to get the most out of this book.
▷Section 1: Bagging and Boosting
-Chapter 1: Machine Learning Landscape
-Chapter 2: Decision Trees in Depth
-Chapter 3: Bagging with Random Forests
-Chapter 4: From Gradient Boosting to XGBoost
▷Section 2: XGBoost
-Chapter 5: XGBoost Unveiled
-Chapter 6: XGBoost Hyperparameters
-Chapter 7: Discovering Exoplanets with XGBoost
▷Section 3: Advanced XGBoost
-Chapter 8: XGBoost Alternative Base Learners
-Chapter 9: XGBoost Kaggle Masters
-Chapter 10: XGBoost Model Deployment
▶What this book covers
- Chapter 1, Machine Learning Landscape, presents XGBoost within the general context of machine learning by introducing linear regression and logistic regression before comparing results with XGBoost. pandas is introduced to preprocess raw data for machine learning by converting categorical columns and clearing null values in a variety of ways.
- Chapter 2, Decision Trees in Depth, presents a detailed examination of decision tree hyperparameters that are used by XGBoost, along with a graphical and statistical analysis of variance and bias that highlights the importance of overfitting, a theme touched on throughout the book.
- Chapter 3, Bagging with Random Forests, presents a general survey of random forests as an XGBoost competitor with a focus on bagging. Additional XGBoost hyperparameters shared with random forests such as n_esimtators and subsample are thoroughly covered.
- Chapter 4, From Gradient Boosting to XGBoost, covers boosting fundamentals, building a booster from scratch in scikit-learn, fine-tuning new XGBoost hyperparameters such as eta, and comparing runtimes between gradient boosting and XGBoost to highlight XGBoost's impressive speed.
- Chapter 5, XGBoost Unveiled, analyzes the mathematical derivations of XGBoost algorithms and features a historically relevant case study featuring XGBoost's role as the winning model in the Higgs Boson Kaggle Competition. Standard XGBoost parameters are discussed, base models are built, and the original Python API is covered.
- Chapter 6, XGBoost Hyperparameters, covers all essential XGBoost hyperparameters, summarizes previous tree ensemble hyperparameters, and uses original grid search functions to fine-tune XGBoost models to optimize scores.
- Chapter 7, Discovering Exoplanets with XGBoost, gives you the opportunity to discover exoplanets with XGBoost in a top-to-bottom case study. The pitfalls of imbalanced datasets are analyzed with the confusion matrix and classification report lea
▶ Preface
XGBoost is an industry-proven, open-source software library that provides a gradient boosting framework for scaling billions of data points quickly and efficiently.
The book introduces machine learning and XGBoost in scikit-learn before building up to the theory behind gradient boosting. You'll cover decision trees and analyze bagging in the machine learning context, learning hyperparameters that extend to XGBoost along the way. You'll build gradient boosting models from scratch and extend gradient boosting to big data while recognizing speed limitations using timers. Details in XGBoost are explored with a focus on speed enhancements and deriving parameters mathematically. With the help of detailed case studies, you'll practice building and fine-tuning XGBoost classifiers and regressors using scikit-learn and the original Python API. You'll leverage XGBoost hyperparameters to improve scores, correct missing values, scale imbalanced datasets, and fine-tune alternative base learners. Finally, you'll apply advanced XGBoost techniques like building non-correlated ensembles, stacking models, and preparing models for industry deployment using sparse matrices, customized transformers, and pipelines.
By the end of the book, you'll be able to build high-performing machine learning models using XGBoost with minimal errors and maximum speed.
작가정보
저자(글) Corey Wade
Corey Wade, M.S. Mathematics, M.F.A. Writing and Consciousness, is the founder and director of Berkeley Coding Academy, where he teaches machine learning and AI to teens from all over the world. Additionally, Corey chairs the Math Department at the Independent Study Program of Berkeley High School, where he teaches programming and advanced math. His additional experience includes teaching natural language processing with Hello World, developing data science curricula with Pathstream, and publishing original statistics (3NG) and machine learning articles with Towards Data Science, Springboard, and Medium. Corey is co-author of the Python Workshop, also published by Packt.
이 상품의 총서
Klover리뷰 (0)
- - e교환권은 적립일로부터 180일 동안 사용 가능합니다.
- - 리워드는 5,000원 이상 eBook, 오디오북, 동영상에 한해 다운로드 완료 후 리뷰 작성 시 익일 제공됩니다. (2024년 9월 30일부터 적용)
- - 리워드는 한 상품에 최초 1회만 제공됩니다.
- - sam 이용권 구매 상품 / 선물받은 eBook은 리워드 대상에서 제외됩니다.
- 도서나 타인에 대해 근거 없이 비방을 하거나 타인의 명예를 훼손할 수 있는 리뷰
- 도서와 무관한 내용의 리뷰
- 인신공격이나 욕설, 비속어, 혐오 발언이 개재된 리뷰
- 의성어나 의태어 등 내용의 의미가 없는 리뷰
구매 후 리뷰 작성 시, e교환권 100원 적립
문장수집
- 구매 후 90일 이내에 문장 수집 등록 시 e교환권 100원을 적립해 드립니다.
- e교환권은 적립일로부터 180일 동안 사용 가능합니다.
- 리워드는 5,000원 이상 eBook에 한해 다운로드 완료 후 문장수집 등록 시 제공됩니다. (2024년 9월 30일부터 적용)
- 리워드는 한 상품에 최초 1회만 제공됩니다.
- sam 이용권 구매 상품 / 선물받은 eBook / 오디오북·동영상 상품/주문취소/환불 시 리워드 대상에서 제외됩니다.
구매 후 문장수집 작성 시, e교환권 100원 적립
신규가입 혜택 지급이 완료 되었습니다.
바로 사용 가능한 교보e캐시 1,000원 (유효기간 7일)
지금 바로 교보eBook의 다양한 콘텐츠를 이용해 보세요!
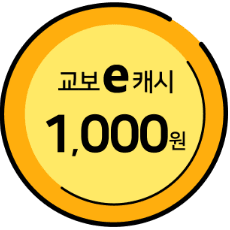
- 구매 후 90일 이내 작성 시, e교환권 100원 (최초1회)
- 리워드 제외 상품 : 마이 > 라이브러리 > Klover리뷰 > 리워드 안내 참고
- 콘텐츠 다운로드 또는 바로보기 완료 후 리뷰 작성 시 익일 제공
가장 와 닿는 하나의 키워드를 선택해주세요.
총 5MB 이하로 jpg,jpeg,png 파일만 업로드 가능합니다.
신고 사유를 선택해주세요.
신고 내용은 이용약관 및 정책에 의해 처리됩니다.
허위 신고일 경우, 신고자의 서비스 활동이 제한될 수
있으니 유의하시어 신중하게 신고해주세요.
이 글을 작성한 작성자의 모든 글은 블라인드 처리 됩니다.
구매 후 90일 이내 작성 시, e교환권 100원 적립
eBook 문장수집은 웹에서 직접 타이핑 가능하나, 모바일 앱에서 도서를 열람하여 문장을 드래그하시면 직접 타이핑 하실 필요 없이 보다 편하게 남길 수 있습니다.
차감하실 sam이용권을 선택하세요.
차감하실 sam이용권을 선택하세요.
선물하실 sam이용권을 선택하세요.
-
보유 권수 / 선물할 권수0권 / 1권
-
받는사람 이름받는사람 휴대전화
- 구매한 이용권의 대한 잔여권수를 선물할 수 있습니다.
- 열람권은 1인당 1권씩 선물 가능합니다.
- 선물한 열람권이 ‘미등록’ 상태일 경우에만 ‘열람권 선물내역’화면에서 선물취소 가능합니다.
- 선물한 열람권의 등록유효기간은 14일 입니다.
(상대방이 기한내에 등록하지 않을 경우 소멸됩니다.) - 무제한 이용권일 경우 열람권 선물이 불가합니다.
첫 구매 시 교보e캐시 지급해 드립니다.
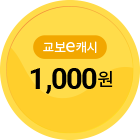
- 첫 구매 후 3일 이내 다운로드 시 익일 자동 지급
- 한 ID당 최초 1회 지급 / sam 이용권 제외
- 구글바이액션을 통해 교보eBook 구매 이력이 없는 회원 대상
- 교보e캐시 1,000원 지급 (유효기간 지급일로부터 7일)