Modern Computer Vision with PyTorch
2020년 11월 27일 출간
- eBook 상품 정보
- 파일 정보 pdf (78.95MB)
- ISBN 9781839216534
- 쪽수 805쪽
- 지원기기 교보eBook App, PC e서재, 리더기, 웹뷰어
-
교보eBook App
듣기(TTS) 불가능
TTS 란?텍스트를 음성으로 읽어주는 기술입니다.
- 전자책의 편집 상태에 따라 본문의 흐름과 다르게 텍스트를 읽을 수 있습니다.
- 전자책 화면에 표기된 주석 등을 모두 읽어 줍니다.
- 이미지 형태로 제작된 전자책 (예 : ZIP 파일)은 TTS 기능을 지원하지 않습니다.
- '교보 ebook' 앱을 최신 버전으로 설치해야 이용 가능합니다. (Android v3. 0.26, iOS v3.0.09,PC v1.2 버전 이상)
PDF 필기 Android 가능 (iOS예정)
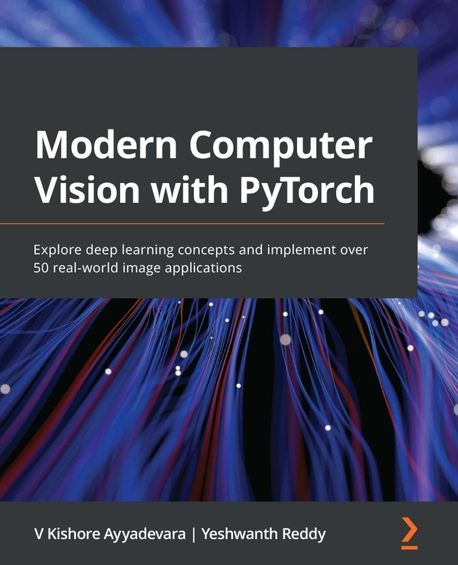
쿠폰적용가 31,500원
10% 할인 | 5%P 적립이 상품은 배송되지 않는 디지털 상품이며,
교보eBook앱이나 웹뷰어에서 바로 이용가능합니다.
카드&결제 혜택
- 5만원 이상 구매 시 추가 2,000P
- 3만원 이상 구매 시, 등급별 2~4% 추가 최대 416P
- 리뷰 작성 시, e교환권 추가 최대 300원
작품소개
이 상품이 속한 분야
▶Book Description
Deep learning is the driving force behind many recent advances in various computer vision (CV) applications. This book takes a hands-on approach to help you to solve over 50 CV problems using PyTorch1.x on real-world datasets.
You'll start by building a neural network (NN) from scratch using NumPy and PyTorch and discover best practices for tweaking its hyperparameters. You'll then perform image classification using convolutional neural networks and transfer learning and understand how they work. As you progress, you'll implement multiple use cases of 2D and 3D multi-object detection, segmentation, human-pose-estimation by learning about the R-CNN family, SSD, YOLO, U-Net architectures, and the Detectron2 platform. The book will also guide you in performing facial expression swapping, generating new faces, and manipulating facial expressions as you explore autoencoders and modern generative adversarial networks. You'll learn how to combine CV with NLP techniques, such as LSTM and transformer, and RL techniques, such as Deep Q-learning, to implement OCR, image captioning, object detection, and a self-driving car agent. Finally, you'll move your NN model to production on the AWS Cloud.
By the end of this book, you'll be able to leverage modern NN architectures to solve over 50 real-world CV problems confidently.
▶What You Will Learn
-Train a NN from scratch with NumPy and PyTorch
-Implement 2D and 3D multi-object detection and segmentation
-Generate digits and DeepFakes with autoencoders and advanced GANs
-Manipulate images using CycleGAN, Pix2PixGAN, StyleGAN2, and SRGAN
-Combine CV with NLP to perform OCR, image captioning, and object detection
-Combine CV with reinforcement learning to build agents that play pong and self-drive a car
-Deploy a deep learning model on the AWS server using FastAPI and Docker
-Implement over 35 NN architectures and common OpenCV utilities
▶Key Features
-Implement solutions to 50 real-world computer vision applications using PyTorch
-Understand the theory and working mechanisms of neural network architectures and their implementation
-Discover best practices using a custom library created especially for this book
▶Who This Book Is For
This book is for beginners to PyTorch and intermediate-level machine learning practitioners who are looking to get well-versed with computer vision techniques using deep learning and PyTorch. If you are just getting started with neural networks, you'll find the use cases accompanied by notebooks in GitHub present in this book useful. Basic knowledge of the Python programming language and machine learning is all you need to get started with this book.
▷Section 1 - Fundamentals of Deep Learning for Computer Vision
-Chapter 1: Artificial Neural Network Fundamentals
-Chapter 2: PyTorch Fundamentals
-Chapter 3: Building a Deep Neural Network with PyTorch
▷Section 2 - Object Classification and Detection
-Chapter 4: Introducing Convolutional Neural Networks
-Chapter 5: Transfer Learning for Image Classification
-Chapter 6: Practical Aspects of Image Classification
-Chapter 7: Basics of Object Detection
-Chapter 8: Advanced Object Detection
-Chapter 9: Image Segmentation
-Chapter 10: Applications of Object Detection and Segmentation
▷Section 3 - Image Manipulation
-Chapter 11: Autoencoders and Image Manipulation
-Chapter 12: Image Generation Using GANs
-Chapter 13: Advanced GANs to Manipulate Images
▷Section 4 - Combining Computer Vision with Other Techniques
-Chapter 14: Training with Minimal Data Points
-Chapter 15: Combining Computer Vision and NLP Techniques
-Chapter 16: Combining Computer Vision and Reinforcement Learning
-Chapter 17: Moving a Model to Production
-Chapter 18: Using OpenCV Utilities for Image Analysis
▶What this book covers
- Chapter 1, Artificial Neural Network Fundamentals, gives you the complete details of how a neural network works. You will start by learning the key terminology associated with neural networks. Next, you will understand the working details of the building blocks and build a neural network from scratch on a toy dataset. By the end of this chapter, you will be confident about how a neural network works.
- Chapter 2, PyTorch Fundamentals, introduces you to working with PyTorch. You will learn about the ways of creating and manipulating tensor objects before learning about the different ways of building a neural network model using PyTorch. You will still work with a toy dataset so that you understand the specifics of working with PyTorch.
- Chapter 3, Building a Deep Neural Network with PyTorch, combines all that has been covered in the previous chapters to understand the impact of various neural network hyperparameters on model accuracy. By the end of this chapter, you will be confident about working with neural networks on a realistic dataset.
- Chapter 4, Introducing Convolutional Neural Networks, details the challenges of using a vanilla neural network and you will be exposed to the reason why convolutional neural networks overcome the various limitations of traditional neural networks. You will dive deep into the working details of CNN and understand the various components in it. Next, you will learn the best practices of working with images. In this chapter, you will start working with real-world images and learn the intricacies
of how CNNs help in image classification.
- Chapter 5, Transfer Learning for Image Classification, exposes you to solving image classification problems in real-world. You will learn about multiple transfer learning architectures and also understand how it helps in significantly improving the image classification accuracy. Next, you will leverage transfer learning to implement the use cases of facial keypoint detection and age, gender estimation.
- Chapter 6, Practical Aspects of Image Classification, provides insight into the practical aspects to take care of while building and deploying image classification models. You will practically see the advantages of leveraging data augmentation and batch normalization on real-world data. Further, you will learn about how class activation maps help in explaining the reason why CNN model predicted a certain outcome. By the end of this chapter, you can confidently tackle a majority of image classification problems and leverage the models discussed in the previous 3 chapters on your custom dataset.
- Chapter 7, Basics of Object Detection, lays the foundation for object detection where you will learn about the various techniques that are used to build an object detection model. Next, you will learn about region proposal-based object-detection techniques through a use case where you will implement a model to locate trucks and buses in an image.
- Chapter 8, Advanced Object Detection, exposes you to the limitations of the regionproposal based architectures. You will then learn about the working details of more advanced architectures that address the issues of region proposal-based architectures. You will implement all the architectures on the same dataset (trucks vs buses detection) so that you can contrast how each architecture works.
- Chapter 9, Image Segmentation, builds upon the learnings in previous chapters and will help you build models that pin-point the location of the objects of various classes as well as instances of objects in an image. You will implement the use cases on images of a road and also on images of common household. By the end of this chapter, you will confidently tackle any image classification, object detection/ segmentation problem and solve it by building a model using PyTorch.
...
▶ Preface
Artificial Intelligence (AI) is here, and has become a powerful force and is fuelling some of the modern applications that are used on a daily basis. Much like the discovery/invention of fire, wheel, oil, electricity, and electronics - Artificial Intelligence is reshaping our world in ways that we could only fantasize about. AI has been historically a niche computer science subject, offered by a handful of labs. But because of the explosion of excellent theory, increase in computing power, and availability of data, the field started growing exponentially since the 2000s and has shown no sign of slowing down anytime soon.
AI has proven again and again that given the right algorithm and enough amount of data, it can learn the task by itself with limited human intervention and produce results that rival human judgement and sometimes even surpass them. Whether you are a rookie learning the ropes or a veteran driving large organizations, there is every reason to understand how AI works. Neural networks are some of the most flexible classes of Artificial Intelligence algorithms that have been adapted to a vast range of applications including structured data, text, and vision domains.
This book starts with the basics of neural networks and covers over 50 applications of computer vision. First, you will build a neural network (NN) from scratch using both NumPy, PyTorch, and then learn the best practices of tweaking a NN's hyperparameters. As we progress, you will learn about CNNs, transfer-learning with a focus on classifying images. You will also learn about the practical aspects to take care of while building a NN model.
Next, you will learn about multi-object detection, segmentation, and implement them using R-CNN family, SSD, YOLO, U-Net, Mask-RCNN architectures. You will then learn to use the Detectron2 framework to simplify the process of building a NN for object detection and human-pose-estimation. Finally, you will implement 3-D object detection.
Subsequently, you will learn about auto-encoders and GANs with a strong focus on image manipulation and generation. Here, you will implement VAE, DCGAN, CGAN, Pix2Pix, CycleGan, StyleGAN2, SRGAN, Style-Transfer to manipulate images on a variety of tasks.
You will then learn to combine NLP and CV techniques while performing OCR, Image Captioning, object detection with transformers. Next, you will learn to combine RL with CV techniques to implement a self-driving car agent. Finally, you'll wrap up with moving a NN model to production and learn conventional CV techniques using the OpenCV library.
작가정보
저자(글) V Kishore Ayyadevara
V Kishore Ayyadevara leads a team focused on using AI to solve problems in the healthcare space. He has more than 10 years' experience in the field of data science with prominent technology companies. In his current role, he is responsible for developing a variety of cutting-edge analytical solutions that have an impact at scale while building strong technical teams.
저자(글) Yeshwanth Reddy
Yeshwanth Reddy is a senior data scientist with a strong focus on the research and implementation of cutting-edge technologies to solve problems in the health and computer vision domains. He has filed four patents in the field of OCR. He also has 2 years of teaching experience, where he delivered sessions to thousands of students in the fields of statistics, machine learning, AI, and natural language processing. He has completed his MTech and BTech at IIT Madras.
이 상품의 총서
Klover리뷰 (0)
- - e교환권은 적립일로부터 180일 동안 사용 가능합니다.
- - 리워드는 1,000원 이상 eBook, 오디오북, 동영상에 한해 다운로드 완료 후 리뷰 작성 시 익일 제공됩니다.
- - 리워드는 한 상품에 최초 1회만 제공됩니다.
- - sam 이용권 구매 상품 / 선물받은 eBook은 리워드 대상에서 제외됩니다.
- 도서나 타인에 대해 근거 없이 비방을 하거나 타인의 명예를 훼손할 수 있는 리뷰
- 도서와 무관한 내용의 리뷰
- 인신공격이나 욕설, 비속어, 혐오 발언이 개재된 리뷰
- 의성어나 의태어 등 내용의 의미가 없는 리뷰
구매 후 리뷰 작성 시, e교환권 100원 적립
문장수집
- 구매 후 90일 이내에 문장 수집 등록 시 e교환권 100원을 적립해 드립니다.
- e교환권은 적립일로부터 180일 동안 사용 가능합니다.
- 리워드는 1,000원 이상 eBook에 한해 다운로드 완료 후 문장수집 등록 시 제공됩니다.
- 리워드는 한 상품에 최초 1회만 제공됩니다.
- sam 이용권 구매 상품/오디오북·동영상 상품/주문취소/환불 시 리워드 대상에서 제외됩니다.
구매 후 문장수집 작성 시, e교환권 100원 적립
신규가입 혜택 지급이 완료 되었습니다.
바로 사용 가능한 교보e캐시 1,000원 (유효기간 7일)
지금 바로 교보eBook의 다양한 콘텐츠를 이용해 보세요!
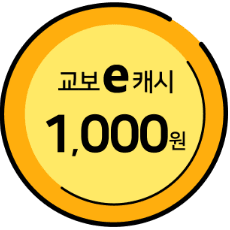
- 구매 후 90일 이내 작성 시, e교환권 100원 (최초1회)
- 리워드 제외 상품 : 마이 > 라이브러리 > Klover리뷰 > 리워드 안내 참고
- 콘텐츠 다운로드 또는 바로보기 완료 후 리뷰 작성 시 익일 제공
가장 와 닿는 하나의 키워드를 선택해주세요.
총 5MB 이하로 jpg,jpeg,png 파일만 업로드 가능합니다.
신고 사유를 선택해주세요.
신고 내용은 이용약관 및 정책에 의해 처리됩니다.
허위 신고일 경우, 신고자의 서비스 활동이 제한될 수
있으니 유의하시어 신중하게 신고해주세요.
이 글을 작성한 작성자의 모든 글은 블라인드 처리 됩니다.
구매 후 90일 이내 작성 시, e교환권 100원 적립
eBook 문장수집은 웹에서 직접 타이핑 가능하나, 모바일 앱에서 도서를 열람하여 문장을 드래그하시면 직접 타이핑 하실 필요 없이 보다 편하게 남길 수 있습니다.
차감하실 sam이용권을 선택하세요.
차감하실 sam이용권을 선택하세요.
선물하실 sam이용권을 선택하세요.
-
보유 권수 / 선물할 권수0권 / 1권
-
받는사람 이름받는사람 휴대전화
- 구매한 이용권의 대한 잔여권수를 선물할 수 있습니다.
- 열람권은 1인당 1권씩 선물 가능합니다.
- 선물한 열람권이 ‘미등록’ 상태일 경우에만 ‘열람권 선물내역’화면에서 선물취소 가능합니다.
- 선물한 열람권의 등록유효기간은 14일 입니다.
(상대방이 기한내에 등록하지 않을 경우 소멸됩니다.) - 무제한 이용권일 경우 열람권 선물이 불가합니다.
첫 구매 시 교보e캐시 지급해 드립니다.
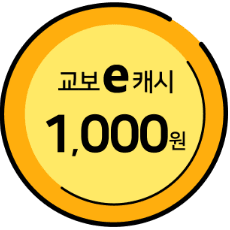
- 첫 구매 후 3일 이내 다운로드 시 익일 자동 지급
- 한 ID당 최초 1회 지급 / sam 이용권 제외
- 구글북액션을 통해 교보eBook 구매 이력이 없는 회원 대상
- 교보e캐시 1,000원 지급 (유효기간 지급일로부터 7일)