Deep Reinforcement Learning Hands-On Second Edition
2020년 01월 31일 출간
- eBook 상품 정보
- 파일 정보 pdf (15.27MB)
- ISBN 9781838820046
- 쪽수 826쪽
- 지원기기 교보eBook App, PC e서재, 리더기, 웹뷰어
-
교보eBook App
듣기(TTS) 불가능
TTS 란?텍스트를 음성으로 읽어주는 기술입니다.
- 전자책의 편집 상태에 따라 본문의 흐름과 다르게 텍스트를 읽을 수 있습니다.
- 전자책 화면에 표기된 주석 등을 모두 읽어 줍니다.
- 이미지 형태로 제작된 전자책 (예 : ZIP 파일)은 TTS 기능을 지원하지 않습니다.
- '교보 ebook' 앱을 최신 버전으로 설치해야 이용 가능합니다. (Android v3. 0.26, iOS v3.0.09,PC v1.2 버전 이상)
PDF 필기 Android 가능 (iOS예정)
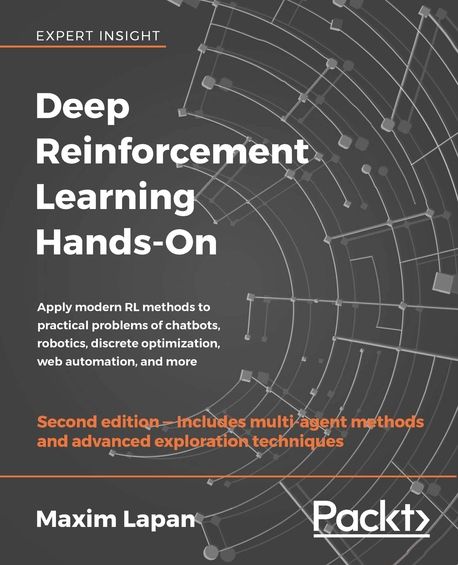
쿠폰적용가 22,500원
10% 할인 | 5%P 적립이 상품은 배송되지 않는 디지털 상품이며,
교보eBook앱이나 웹뷰어에서 바로 이용가능합니다.
카드&결제 혜택
- 5만원 이상 구매 시 추가 2,000P
- 3만원 이상 구매 시, 등급별 2~4% 추가 최대 416P
- 리뷰 작성 시, e교환권 추가 최대 300원
작품소개
이 상품이 속한 분야
Deep Reinforcement Learning Hands-On, Second Edition is an updated and expanded version of the bestselling guide to the very latest reinforcement learning (RL) tools and techniques. It provides you with an introduction to the fundamentals of RL, along with the hands-on ability to code intelligent learning agents to perform a range of practical tasks.
With six new chapters devoted to a variety of up-to-the-minute developments in RL, including discrete optimization (solving the Rubik's Cube), multi-agent methods, Microsoft's TextWorld environment, advanced exploration techniques, and more, you will come away from this book with a deep understanding of the latest innovations in this emerging field.
In addition, you will gain actionable insights into such topic areas as deep Q-networks, policy gradient methods, continuous control problems, and highly scalable, non-gradient methods. You will also discover how to build a real hardware robot trained with RL for less than $100 and solve the Pong environment in just 30 minutes of training using step-by-step code optimization.
In short, Deep Reinforcement Learning Hands-On, Second Edition, is your companion to navigating the exciting complexities of RL as it helps you attain experience and knowledge through real-world examples.
▶What You Will Learn
- Understand the deep learning context of RL and implement complex deep learning models
- Evaluate RL methods including cross-entropy, DQN, actor-critic, TRPO, PPO, DDPG, D4PG, and others
- Build a practical hardware robot trained with RL methods for less than $100
- Discover Microsoft's TextWorld environment, which is an interactive fiction games platform
- Use discrete optimization in RL to solve a Rubik's Cube
- Teach your agent to play Connect 4 using AlphaGo Zero
- Explore the very latest deep RL research on topics including AI chatbots
- Discover advanced exploration techniques, including noisy networks and network distillation techniques
▶Key Features
- Second edition of the bestselling introduction to deep reinforcement learning, expanded with six new chapters
- Learn advanced exploration techniques including noisy networks, pseudo-count, and network distillation methods
- Apply RL methods to cheap hardware robotics platforms
▶Who This Book Is For
Some fluency in Python is assumed. Sound understanding of the fundamentals of deep learning will be helpful. This book is an introduction to deep RL and requires no background in RL
1. What Is Reinforcement Learning?
2. OpenAI Gym
3. Deep Learning with PyTorch
4. The Cross-Entropy Method
5. Tabular Learning and the Bellman Equation
6. Deep Q-Networks
7. Higher-Level RL Libraries
8. DQN Extensions
9. Ways to Speed up RL
10. Stocks Trading Using RL
11. Policy Gradients ? an Alternative
12. The Actor-Critic Method
13. Asynchronous Advantage Actor-Critic
14. Training Chatbots with RL
15. The TextWorld Environment
16. Web Navigation
17. Continuous Action Space
18. RL in Robotics
19. Trust Regions ? PPO, TRPO, ACKTR, and SAC
20. Black-Box Optimization in RL
21. Advanced Exploration
22. Beyond Model-Free ? Imagination
23. AlphaGo Zero
24. RL in Discrete Optimization
25. Multi-agent RL
▶What this book covers
- Chapter 1, What Is Reinforcement Learning?, contains an introduction to RL ideas and the main formal models.
- Chapter 2, OpenAI Gym, introduces the practical aspects of RL, using the open source library Gym.
- Chapter 3, Deep Learning with PyTorch, gives a quick overview of the PyTorch library.
- Chapter 4, The Cross-Entropy Method, introduces one of the simplest methods in RL to give you an impression of RL methods and problems.
- Chapter 5, Tabular Learning and the Bellman Equation, introduces the value-based family of RL methods.
- Chapter 6, Deep Q-Networks, describes deep Q-networks (DQNs), an extension of the basic value-based methods, allowing us to solve a complicated environment.
- Chapter 7, Higher-Level RL Libraries, describes the library PTAN, which we will use in the book to simplify the implementations of RL methods.
- Chapter 8, DQN Extensions, gives a detailed overview of a modern extension to the DQN method, to improve its stability and convergence in complex environments.
- Chapter 9, Ways to Speed up RL Methods, provides an overview of ways to make the execution of RL code faster.
- Chapter 10, Stocks Trading Using RL, is the first practical project and focuses on applying the DQN method to stock trading.
- Chapter 11, Policy Gradients―an Alternative, introduces another family of RL methods that is based on policy learning.
- Chapter 12, The Actor-Critic Method, describes one of the most widely used methods in RL.
- Chapter 13, Asynchronous Advantage Actor-Critic, extends the actor-critic method with parallel environment communication, which improves stability and convergence.
- Chapter 14, Training Chatbots with RL, is the second project and shows how to apply RL methods to natural language processing problems.
- Chapter 15, The TextWorld Environment, covers the application of RL methods to interactive fiction games.
- Chapter 16, Web Navigation, is another long project that applies RL to web page navigation using the MiniWoB set of tasks.
- Chapter 17, Continuous Action Space, describes the specifics of environments using continuous action spaces and various methods.
- Chapter 18, RL in Robotics, covers the application of RL methods to robotics problems. In this chapter, I describe the process of building and training a small hardware robot with RL methods.
- Chapter 19, Trust Regions ? PPO, TRPO, ACKTR, and SAC, is yet another chapter about continuous action spaces describing the trust region set of methods.
- Chapter 20, Black-Box Optimization in RL, shows another set of methods that don't use gradients in their explicit form.
- Chapter 21, Advanced Exploration, covers different approaches that can be used for better exploration of the environment.
- Chapter 22, Beyond Model-Free ? Imagination, introduces the model-based approach to RL and uses recent research results about imagination in RL.
- Chapter 23, AlphaGo Zero, describes the AlphaGo Zero method and applies it to the game Connect 4.
- Chapter 24, RL in Discrete Optimization, describes the application of RL methods to the domain of discrete optimization, using the Rubik's Cube as an environment.
- Chapter 25, Multi-agent RL, introduces a relatively new direction of RL methods for situations with multiple agents.
▶ Preface
The topic of this book is reinforcement learning (RL), which is a subfield of machine learning (ML); it focuses on the general and challenging problem of learning optimal behavior in a complex environment. The learning process is driven only by the reward value and observations obtained from the environment. This model is very general and can be applied to many practical situations, from playing games to optimizing complex manufacturing processes.
Due to its flexibility and generality, the field of RL is developing very quickly and attracting lots of attention, both from researchers who are trying to improve existing methods or create new methods and from practitioners interested in solving their problems in the most efficient way.
작가정보
저자(글) Maxim Lapan
Maxim Lapan is a deep learning enthusiast and independent researcher. His background and 15 years' work expertise as a software developer and a systems architect lies from low-level Linux kernel driver development to performance optimization and design of distributed applications working on thousands of servers. With vast work experiences in big data, machine learning, and large parallel distributed HPC and non-HPC systems, he is able to explain a number of complicated concepts in simple words and vivid examples. His current areas of interest are in practical applications of deep learning, such as deep natural language processing and deep reinforcement learning. Maxim lives in Moscow, Russian Federation, with his family.
이 상품의 총서
Klover리뷰 (0)
- - e교환권은 적립일로부터 180일 동안 사용 가능합니다.
- - 리워드는 1,000원 이상 eBook, 오디오북, 동영상에 한해 다운로드 완료 후 리뷰 작성 시 익일 제공됩니다.
- - 리워드는 한 상품에 최초 1회만 제공됩니다.
- - sam 이용권 구매 상품 / 선물받은 eBook은 리워드 대상에서 제외됩니다.
- 도서나 타인에 대해 근거 없이 비방을 하거나 타인의 명예를 훼손할 수 있는 리뷰
- 도서와 무관한 내용의 리뷰
- 인신공격이나 욕설, 비속어, 혐오 발언이 개재된 리뷰
- 의성어나 의태어 등 내용의 의미가 없는 리뷰
구매 후 리뷰 작성 시, e교환권 100원 적립
문장수집
- 구매 후 90일 이내에 문장 수집 등록 시 e교환권 100원을 적립해 드립니다.
- e교환권은 적립일로부터 180일 동안 사용 가능합니다.
- 리워드는 1,000원 이상 eBook에 한해 다운로드 완료 후 문장수집 등록 시 제공됩니다.
- 리워드는 한 상품에 최초 1회만 제공됩니다.
- sam 이용권 구매 상품/오디오북·동영상 상품/주문취소/환불 시 리워드 대상에서 제외됩니다.
구매 후 문장수집 작성 시, e교환권 100원 적립
신규가입 혜택 지급이 완료 되었습니다.
바로 사용 가능한 교보e캐시 1,000원 (유효기간 7일)
지금 바로 교보eBook의 다양한 콘텐츠를 이용해 보세요!
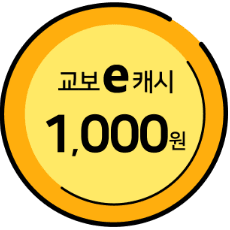
- 구매 후 90일 이내 작성 시, e교환권 100원 (최초1회)
- 리워드 제외 상품 : 마이 > 라이브러리 > Klover리뷰 > 리워드 안내 참고
- 콘텐츠 다운로드 또는 바로보기 완료 후 리뷰 작성 시 익일 제공
가장 와 닿는 하나의 키워드를 선택해주세요.
총 5MB 이하로 jpg,jpeg,png 파일만 업로드 가능합니다.
신고 사유를 선택해주세요.
신고 내용은 이용약관 및 정책에 의해 처리됩니다.
허위 신고일 경우, 신고자의 서비스 활동이 제한될 수
있으니 유의하시어 신중하게 신고해주세요.
이 글을 작성한 작성자의 모든 글은 블라인드 처리 됩니다.
구매 후 90일 이내 작성 시, e교환권 100원 적립
eBook 문장수집은 웹에서 직접 타이핑 가능하나, 모바일 앱에서 도서를 열람하여 문장을 드래그하시면 직접 타이핑 하실 필요 없이 보다 편하게 남길 수 있습니다.
차감하실 sam이용권을 선택하세요.
차감하실 sam이용권을 선택하세요.
선물하실 sam이용권을 선택하세요.
-
보유 권수 / 선물할 권수0권 / 1권
-
받는사람 이름받는사람 휴대전화
- 구매한 이용권의 대한 잔여권수를 선물할 수 있습니다.
- 열람권은 1인당 1권씩 선물 가능합니다.
- 선물한 열람권이 ‘미등록’ 상태일 경우에만 ‘열람권 선물내역’화면에서 선물취소 가능합니다.
- 선물한 열람권의 등록유효기간은 14일 입니다.
(상대방이 기한내에 등록하지 않을 경우 소멸됩니다.) - 무제한 이용권일 경우 열람권 선물이 불가합니다.
첫 구매 시 교보e캐시 지급해 드립니다.
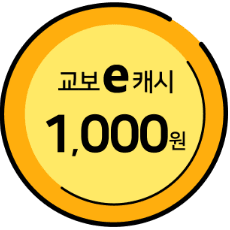
- 첫 구매 후 3일 이내 다운로드 시 익일 자동 지급
- 한 ID당 최초 1회 지급 / sam 이용권 제외
- 구글북액션을 통해 교보eBook 구매 이력이 없는 회원 대상
- 교보e캐시 1,000원 지급 (유효기간 지급일로부터 7일)