Deep Learning with R for Beginners
2019년 05월 20일 출간
- eBook 상품 정보
- 파일 정보 pdf (17.68MB)
- ISBN 9781838647223
- 쪽수 606쪽
- 지원기기 교보eBook App, PC e서재, 리더기, 웹뷰어
-
교보eBook App
듣기(TTS) 가능
TTS 란?텍스트를 음성으로 읽어주는 기술입니다.
- 전자책의 편집 상태에 따라 본문의 흐름과 다르게 텍스트를 읽을 수 있습니다.
- 전자책 화면에 표기된 주석 등을 모두 읽어 줍니다.
- 이미지 형태로 제작된 전자책 (예 : ZIP 파일)은 TTS 기능을 지원하지 않습니다.
- '교보 ebook' 앱을 최신 버전으로 설치해야 이용 가능합니다. (Android v3. 0.26, iOS v3.0.09,PC v1.2 버전 이상)
PDF 필기 Android 가능 (iOS예정)
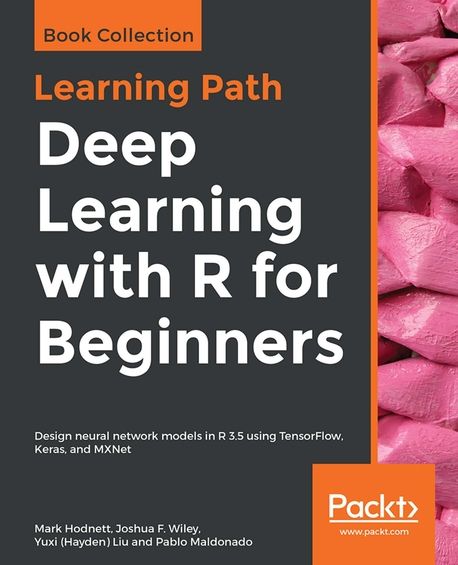
쿠폰적용가 24,300원
10% 할인 | 5%P 적립이 상품은 배송되지 않는 디지털 상품이며,
교보eBook앱이나 웹뷰어에서 바로 이용가능합니다.
카드&결제 혜택
- 5만원 이상 구매 시 추가 2,000P
- 3만원 이상 구매 시, 등급별 2~4% 추가 최대 416P
- 리뷰 작성 시, e교환권 추가 최대 300원
작품소개
이 상품이 속한 분야
- Implement credit card fraud detection with autoencoders
- Train neural networks to perform handwritten digit recognition using MXNet
- Reconstruct images using variational autoencoders
- Explore the applications of autoencoder neural networks in clustering and dimensionality reduction
- Create natural language processing (NLP) models using Keras and TensorFlow in R
- Prevent models from overfitting the data to improve generalizability
- Build shallow neural network prediction models
▶Key Features
- Get to grips with the fundamentals of deep learning and neural networks
- Use R 3.5 and its libraries and APIs to build deep learning models for computer vision and text processing
- Implement effective deep learning systems in R with the help of end-to-end projects
▶Who This Book Is For
This Learning Path is for aspiring data scientists, data analysts, machine learning developers, and deep learning enthusiasts who are well versed in machine learning concepts and are looking to explore the deep learning paradigm using R. A fundamental understanding of R programming and familiarity with the basic concepts of deep learning are necessary to get the most out of this Learning Path.
1. Getting Started with Deep Learning
2. Training a Prediction Model
3. Deep Learning Fundamentals
4. Training Deep Prediction Models
5. Image Classification Using Convolutional Neural Networks
6. Tuning and Optimizing Models
7. Natural Language Processing Using Deep Learning
8. Deep Learning Models Using TensorFlow in R
9. Anomaly Detection and Recommendation Systems
10. Running Deep Learning Models in the Cloud
11. The Next Level in Deep Learning
12. Handwritten Digit Recognition using Convolutional Neural Networks
13. Traffic Signs Recognition for Intelligent Vehicles
14. Fraud Detection with Autoencoders
15. Text Generation using Recurrent Neural Networks
16. Sentiment Analysis with Word Embedding
▶What this book covers
- Chapter 1, Getting Started with Deep Learning, gives an introduction to deep learning and neural networks. It also gives a brief introduction on how to set up your R environment.
- Chapter 2, Training a Prediction Model, begins with building neural network models using the existing packages in R. This chapter also discusses overfitting, which is an issue in most deep learning models
- Chapter 3, Deep Learning Fundamentals, teaches how to build a neural network in R from scratch. We then show how our code relates to MXNet, a deep learning library.
- Chapter 4, Training Deep Prediction Models, looks at activations and introduces the MXNet library. We then build a deep learning prediction model for a real-life example. We will take a raw dataset of transactional data and develop a data pipeline to create a model that predicts which customers will return in the next 14 days.
- Chapter 5, Image Classification Using Convolutional Neural Networks, looks at image classification tasks. First, we will introduce some of the core concepts, such as convolutional and pooling layers, and then we will show how to use these layers to classify images
- Chapter 6, Tuning and Optimizing Models, discusses how to tune and optimize deep learning models. We look at tuning hyperparameters and using data augmentation.
- Chapter 7, Natural Language Processing Using Deep Learning, shows how to use deep learning for Natural Language Processing (NLP) tasks. We show how deep learning algorithms outperform traditional NLP techniques, while also being much easier to develop
- Chapter 8, Deep Learning Models Using TensorFlow in R, looks at using the TensorFlow API in R. We also look at some additional packages available within TensorFlow that make developing TensorFlow models simpler and help in hyperparameter selection.
- Chapter 9, Anomaly Detection and Recommendation Systems, shows how we can use deep learning models to create embeddings that are lower order representations of data. We then show how to use embeddings for anomaly detection and to create a recommendation system.
- Chapter 10, Running Deep Learning Models in the Cloud, covers how to use AWS, Azure, and Google Cloud services to train deep learning models. This chapter shows how to train your models at low-cost in the cloud.
- Chapter 11, The Next Level in Deep Learning, introduces an end-to-end solution for image classification. We take a set of image files, train a model, reuse that model for transfer learning and then show how to deploy that model to production. We also briefly discuss Generative Adversarial Networks (GANs) and reinforcement learning.
- Chapter 12, Handwritten Digit Recognition Using Convolutional Neural Networks, we begin with a recap of logistic regression and multilayer perceptron. We'll solve the problem with these two algorithms. We will then move on to the biologically inspired variants of multilayer perceptron―convolutional neural networks (CNNs).
- Chapter 13, Traffic Sign Recognition for Intelligent Vehicles, explains how to use CNNs for another application―traffic sign detection. We will also cover several important concepts of deep learning in this chapter and get readers familiar with other popular frameworks and libraries, such as Keras and TensorFlow. We will also introduce the dropout technique as a regularization approach and utilize data augmentation techniques to deal with a lack of training data.
- Chapter 14, Fraud Detection with Autoencoders, introduces a type of deep learning model that can be used for anomaly detection. Outliers can be found within a collection of images, a text corpus, or transactional data. We will dive into applications of autoencoders and how they can be used for outlier detection in this domain.
- Chapter 15, Text Generation Using Recurrent Neural Networks, ...
▶ Preface
Deep learning finds practical applications in several domains, while R is the preferred language for designing and deploying deep learning models.
This Learning Path introduces you to the basics of deep learning and even teaches you to build a neural network model from scratch. As you make your way through the chapters, you'll explore deep learning libraries and understand how to create deep learning models for a variety of challenges, right from anomaly detection to recommendation systems. The book will then help you cover advanced topics, such as generative adversarial networks (GANs), transfer learning, and large-scale deep learning in the cloud, in addition to model optimization, overfitting, and data augmentation. Through real-world projects, you'll also get up to speed with training convolutional neural networks (CNNs), recurrent neural networks (RNNs), and long short-term memory networks (LSTMs) in R.
By the end of this Learning Path, you'll be well versed with deep learning and have the skills you need to implement a number of deep learning concepts in your research work or projects.
This Learning Path includes content from the following Packt products:
- R Deep Learning Essentials - Second Edition by Joshua F. Wiley and Mark Hodnett
- R Deep Learning Projects by Yuxi (Hayden) Liu and Pablo Maldonado
작가정보
저자(글) Mark Hodnett
Mark Hodnett is a data scientist with over 20 years of industry experience in software development, business intelligence systems, and data science. He has worked in a variety of industries, including CRM systems, retail loyalty, IoT systems, and accountancy. He holds a master's in data science and an MBA. He works in Cork, Ireland, as a senior data scientist with AltViz.
저자(글) Joshua F. Wiley
Joshua F. Wiley is a lecturer at Monash University, conducting quantitative research on sleep, stress, and health. He earned his Ph.D. from the University of California, Los Angeles and completed postdoctoral training in primary care and prevention. In statistics and data science, Joshua focuses on biostatistics and is interested in reproducible research and graphical displays of data and statistical models. He develops or co-develops a number of R packages including Varian, a package to conduct Bayesian scale-location structural equation models, and MplusAutomation, a popular package that links R to the commercial Mplus software.
저자(글) Yuxi (Hayden) Liu
Yuxi (Hayden) Liu is an experienced data scientist who's focused on developing machine learning and deep learning models and systems. He has worked in a variety of data-driven domains and has applied his machine learning expertise to computational advertising, recommendation, and network anomaly detection. He published five first-authored IEEE transaction and conference papers during his master's research at the University of Toronto. He is an education enthusiast and the author of a series of machine learning books. His first book, the first edition of Python Machine Learning By Example, was a #1 bestseller on Amazon India in 2017 and 2018. His other books include R Deep Learning Projects and Hands-On Deep Learning Architectures with Python published by Packt.
저자(글) Pablo Maldonado
Pablo Maldonado is an applied mathematician and data scientist with a taste for software development since his days of programming BASIC on a Tandy 1000. As an academic and business consultant, he spends a great deal of his time building applied artificial intelligence solutions for text analytics, sensor and transactional data, and reinforcement learning. Pablo earned his Ph.D. in applied mathematics (with focus on mathematical game theory) at the Universite Pierre et Marie Curie in Paris, France.
이 상품의 총서
Klover리뷰 (0)
- - e교환권은 적립일로부터 180일 동안 사용 가능합니다.
- - 리워드는 1,000원 이상 eBook, 오디오북, 동영상에 한해 다운로드 완료 후 리뷰 작성 시 익일 제공됩니다.
- - 리워드는 한 상품에 최초 1회만 제공됩니다.
- - sam 이용권 구매 상품 / 선물받은 eBook은 리워드 대상에서 제외됩니다.
- 도서나 타인에 대해 근거 없이 비방을 하거나 타인의 명예를 훼손할 수 있는 리뷰
- 도서와 무관한 내용의 리뷰
- 인신공격이나 욕설, 비속어, 혐오 발언이 개재된 리뷰
- 의성어나 의태어 등 내용의 의미가 없는 리뷰
구매 후 리뷰 작성 시, e교환권 100원 적립
문장수집
- 구매 후 90일 이내에 문장 수집 등록 시 e교환권 100원을 적립해 드립니다.
- e교환권은 적립일로부터 180일 동안 사용 가능합니다.
- 리워드는 1,000원 이상 eBook에 한해 다운로드 완료 후 문장수집 등록 시 제공됩니다.
- 리워드는 한 상품에 최초 1회만 제공됩니다.
- sam 이용권 구매 상품/오디오북·동영상 상품/주문취소/환불 시 리워드 대상에서 제외됩니다.
구매 후 문장수집 작성 시, e교환권 100원 적립
신규가입 혜택 지급이 완료 되었습니다.
바로 사용 가능한 교보e캐시 1,000원 (유효기간 7일)
지금 바로 교보eBook의 다양한 콘텐츠를 이용해 보세요!
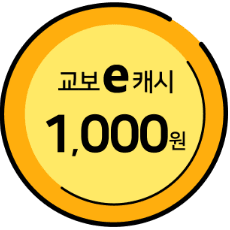
- 구매 후 90일 이내 작성 시, e교환권 100원 (최초1회)
- 리워드 제외 상품 : 마이 > 라이브러리 > Klover리뷰 > 리워드 안내 참고
- 콘텐츠 다운로드 또는 바로보기 완료 후 리뷰 작성 시 익일 제공
가장 와 닿는 하나의 키워드를 선택해주세요.
총 5MB 이하로 jpg,jpeg,png 파일만 업로드 가능합니다.
신고 사유를 선택해주세요.
신고 내용은 이용약관 및 정책에 의해 처리됩니다.
허위 신고일 경우, 신고자의 서비스 활동이 제한될 수
있으니 유의하시어 신중하게 신고해주세요.
이 글을 작성한 작성자의 모든 글은 블라인드 처리 됩니다.
구매 후 90일 이내 작성 시, e교환권 100원 적립
eBook 문장수집은 웹에서 직접 타이핑 가능하나, 모바일 앱에서 도서를 열람하여 문장을 드래그하시면 직접 타이핑 하실 필요 없이 보다 편하게 남길 수 있습니다.
차감하실 sam이용권을 선택하세요.
차감하실 sam이용권을 선택하세요.
선물하실 sam이용권을 선택하세요.
-
보유 권수 / 선물할 권수0권 / 1권
-
받는사람 이름받는사람 휴대전화
- 구매한 이용권의 대한 잔여권수를 선물할 수 있습니다.
- 열람권은 1인당 1권씩 선물 가능합니다.
- 선물한 열람권이 ‘미등록’ 상태일 경우에만 ‘열람권 선물내역’화면에서 선물취소 가능합니다.
- 선물한 열람권의 등록유효기간은 14일 입니다.
(상대방이 기한내에 등록하지 않을 경우 소멸됩니다.) - 무제한 이용권일 경우 열람권 선물이 불가합니다.
첫 구매 시 교보e캐시 지급해 드립니다.
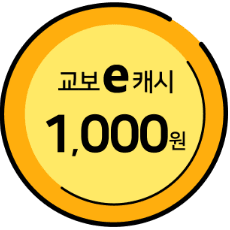
- 첫 구매 후 3일 이내 다운로드 시 익일 자동 지급
- 한 ID당 최초 1회 지급 / sam 이용권 제외
- 구글북액션을 통해 교보eBook 구매 이력이 없는 회원 대상
- 교보e캐시 1,000원 지급 (유효기간 지급일로부터 7일)