Python Deep Learning Second Edition
2019년 01월 16일 출간
- eBook 상품 정보
- 파일 정보 PDF (23.97MB)
- ISBN 9781789349702
- 지원기기 교보eBook App, PC e서재, 리더기, 웹뷰어
-
교보eBook App
듣기(TTS) 가능
TTS 란?텍스트를 음성으로 읽어주는 기술입니다.
- 전자책의 편집 상태에 따라 본문의 흐름과 다르게 텍스트를 읽을 수 있습니다.
- 이미지 형태로 제작된 전자책 (예 : ZIP 파일)은 TTS 기능을 지원하지 않습니다.
PDF 필기가능 (Android, iOS)
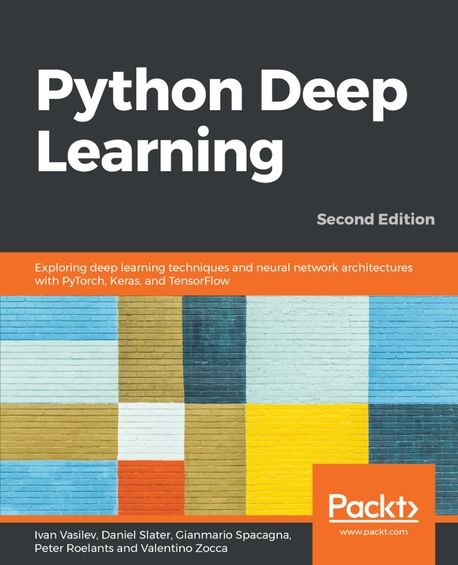
쿠폰적용가 19,800원
10% 할인 | 5%P 적립이 상품은 배송되지 않는 디지털 상품이며,
교보eBook앱이나 웹뷰어에서 바로 이용가능합니다.
카드&결제 혜택
- 5만원 이상 구매 시 추가 2,000P
- 3만원 이상 구매 시, 등급별 2~4% 추가 최대 416P
- 리뷰 작성 시, e교환권 추가 최대 200원
작품소개
이 상품이 속한 분야
? Grasp the mathematical theory behind neural networks and deep learning processes
? Investigate and resolve computer vision challenges using convolutional networks and capsule networks
? Solve generative tasks using variational autoencoders and Generative Adversarial Networks
? Implement complex NLP tasks using recurrent networks (LSTM and GRU) and attention models
? Explore reinforcement learning and understand how agents behave in a complex environment
? Get up to date with applications of deep learning in autonomous vehicles
▶Key Features
? Build a strong foundation in neural networks and deep learning with Python libraries
? Explore advanced deep learning techniques and their applications across computer vision and NLP
? Learn how a computer can navigate in complex environments with reinforcement learning
▶Who This Book Is For
This book is for data science practitioners, machine learning engineers, and those interested in deep learning who have a basic foundation in machine learning and some Python programming experience. A background in mathematics and conceptual understanding of calculus and statistics will help you gain maximum benefit from this book.
1 Machine Learning - an Introduction
2 Neural Networks
3 Deep Learning Fundamentals
4 Computer Vision with Convolutional Networks
5 Advanced Computer Vision
6 Generating Images with GANs and VAEs
7 Recurrent Neural Networks and Language Models
8 Reinforcement Learning Theory
9 Deep Reinforcement Learning for Games
10 Deep Learning in Autonomous Vehicles
▶What this book covers
? Chapter 1, Machine Learning ? an Introduction, will introduce you to the basic ML concepts and terms that we'll be using throughout the book. It will give an overview of the most popular ML algorithms and applications today. It will also introduce the DL library that we'll use throughout the book.
? Chapter 2, Neural Networks, will introduce you to the mathematics of neural networks. We'll learn about their structure, how they make predictions (that's the feedforward part), and how to train them using gradient descent and backpropagation (explained through derivatives). The chapter will also discuss how to represent operations with neural networks as vector operations.
? Chapter 3, Deep Learning Fundamentals, will explain the rationale behind using deep neural networks (as opposed to shallow ones). It will take an overview of the most popular DL libraries and real-world applications of DL.
? Chapter 4, Computer Vision with Convolutional Networks, teaches you about convolutional neural networks (the most popular type of neural network for computer vision tasks). We'll learn about their architecture and building blocks (the convolutional, pooling, and capsule layers) and how to use a convolutional network for an image classification task.
? Chapter 5, Advanced Computer Vision, will build on the previous chapter and cover more advanced computer vision topics. You will learn not only how to classify images, but also how to detect an object's location and segment every pixel of an image. We'll learn about advanced convolutional network architectures and the useful practical technique of transfer learning.
? Chapter 6, Generating Images with GANs and VAEs, will introduce generative models (as opposed to discriminative models, which is what we'll have covered up until this point). You will learn about two of the most popular unsupervised generative model approaches, VAEs and GANs, as well some of their exciting applications.
? Chapter 7, Recurrent Neural Networks and Language Models, will introduce you to the most popular recurrent network architectures: LSTM and gated recurrent unit (GRU). We'll learn about the paradigms of NLP with recurrent neural networks and the latest algorithms and architectures to solve NLP problems. We'll also learn the basics of speech-to-text recognition.
? Chapter 8, Reinforcement Learning Theory, will introduce you to the main paradigms and terms of RL, a separate ML field. You will learn about the most important RL algorithms. We'll also learn about the link between DL and RL. Throughout the chapter, we will use toy examples to better demonstrate the concepts of RL.
? Chapter 9, Deep Reinforcement Learning for Games, you will understand some real-world applications of RL algorithms, such as playing board games and computer games. We'll learn how to combine the knowledge from the previous parts of the book to create betterthan-human computer players on some popular games.
? Chapter 10, Deep Learning in Autonomous vehicles, we'll discuss what sensors autonomous vehicles use, so they can create the 3D model of the environment. These include cameras, radar sensors, ultrasound sensors, Lidar, as well as accurate GPS positioning. We'll talk about how to apply deep learning algorithms for processing the input of these sensors. For example, we can use instance segmentation and object detection to detect pedestrians and vehicles using the vehicle cameras. We'll also make an overview of some of the approaches vehicle manufacturers use to solve this problem (for example Audi, Tesla, and so on).
▶ Preface
With the surge in artificial intelligence in applications catering to both business and consumer needs, deep learning is more important than ever for meeting current and future market demands. With this book, you’ll explore deep learning, and learn how to put machine learning to use in your projects.
This second edition of Python Deep Learning will get you up to speed with deep learning, deep neural networks, and how to train them with high-performance algorithms and popular Python frameworks. You’ll uncover different neural network architectures, such as convolutional networks, recurrent neural networks, long short-term memory (LSTM) networks, and capsule networks. You’ll also learn how to solve problems in the fields of computer vision, natural language processing (NLP), and speech recognition. You'll study generative model approaches such as variational autoencoders and Generative Adversarial Networks (GANs) to generate images. As you delve into newly evolved areas of reinforcement learning, you’ll gain an understanding of state-of-the-art algorithms that are the main components behind popular games Go, Atari, and Dota.
By the end of the book, you will be well-versed with the theory of deep learning along with its real-world applications.
작가정보
저자(글) Ivan Vasilev
Ivan Vasilev started working on the first open source Java Deep Learning library with GPU support in 2013. The library was acquired by a German company, where he continued its development. He has also worked as a machine learning engineer and researcher in the area of medical image classification and segmentation with deep neural networks. Since 2017 he has focused on financial machine learning. He is working on a Python open source algorithmic trading library, which provides the infrastructure to experiment with different ML algorithms. The author holds an MSc degree in Artificial Intelligence from The University of Sofia, St. Kliment Ohridski.
저자(글) Daniel Slater
Daniel Slater started programming at age 11, developing mods for the id Software game Quake. His obsession led him to become a developer working in the gaming industry on the hit computer game series Championship Manager. He then moved into finance, working on risk- and high-performance messaging systems. He now is a staff engineer working on big data at Skimlinks to understand online user behavior. He spends his spare time training AI to beat computer games. He talks at tech conferences about deep learning and reinforcement learning; and the name of his blog is Daniel Slater's blog. His work in this field has been cited by Google.
저자(글) Gianmario Spacagna
Gianmario Spacagna is a senior data scientist at Pirelli, processing sensors and telemetry data for the internet of things (IoT) and connected-vehicle applications. He works closely with tire mechanics, engineers, and business units to analyze and formulate hybrid, physics-driven, and data-driven automotive models. His main expertise is in building ML systems and end-to-end solutions for data products. He holds a master's degree in telematics from the Polytechnic of Turin, as well as one in software engineering of distributed systems from KTH, Stockholm. Prior to Pirelli, he worked in retail and business banking (Barclays), cyber security (Cisco), predictive marketing (AgilOne), and did some occasional freelancing.
저자(글) Peter Roelants
Peter Roelants holds a master's in computer science with a specialization in AI from KU Leuven. He works on applying deep learning to a variety of problems, such as spectral imaging, speech recognition, text understanding, and document information extraction. He currently works at Onfido as a team leader for the data extraction research team, focusing on data extraction from official documents.
저자(글) Valentino Zocca
Valentino Zocca has a PhD degree and graduated with a Laurea in mathematics from the University of Maryland, USA, and University of Rome, respectively, and spent a semester at the University of Warwick. He started working on high-tech projects of an advanced stereo 3D Earth visualization software with head tracking at Autometric, a company later bought by Boeing. There he developed many mathematical algorithms and predictive models, and using Hadoop he automated several satellite-imagery visualization programs. He has worked as an independent consultant at the U.S. Census Bureau, in the USA and in Italy. Currently, Valentino lives in New York and works as an independent consultant to a large financial company.
이 상품의 총서
Klover리뷰 (0)
- - e교환권은 적립일로부터 180일 동안 사용 가능합니다.
- - 리워드는 5,000원 이상 eBook, 오디오북, 동영상에 한해 다운로드 완료 후 리뷰 작성 시 익일 제공됩니다. (2024년 9월 30일부터 적용)
- - 리워드는 한 상품에 최초 1회만 제공됩니다.
- - sam 이용권 구매 상품 / 선물받은 eBook은 리워드 대상에서 제외됩니다.
- 도서나 타인에 대해 근거 없이 비방을 하거나 타인의 명예를 훼손할 수 있는 리뷰
- 도서와 무관한 내용의 리뷰
- 인신공격이나 욕설, 비속어, 혐오 발언이 개재된 리뷰
- 의성어나 의태어 등 내용의 의미가 없는 리뷰
구매 후 리뷰 작성 시, e교환권 100원 적립
문장수집
- 구매 후 90일 이내에 문장 수집 등록 시 e교환권 100원을 적립해 드립니다.
- e교환권은 적립일로부터 180일 동안 사용 가능합니다.
- 리워드는 5,000원 이상 eBook에 한해 다운로드 완료 후 문장수집 등록 시 제공됩니다. (2024년 9월 30일부터 적용)
- 리워드는 한 상품에 최초 1회만 제공됩니다.
- sam 이용권 구매 상품 / 선물받은 eBook / 오디오북·동영상 상품/주문취소/환불 시 리워드 대상에서 제외됩니다.
구매 후 문장수집 작성 시, e교환권 100원 적립
신규가입 혜택 지급이 완료 되었습니다.
바로 사용 가능한 교보e캐시 1,000원 (유효기간 7일)
지금 바로 교보eBook의 다양한 콘텐츠를 이용해 보세요!
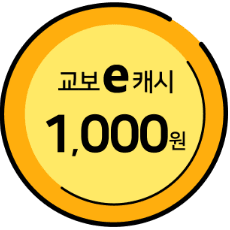
- 구매 후 90일 이내 작성 시, e교환권 100원 (최초1회)
- 리워드 제외 상품 : 마이 > 라이브러리 > Klover리뷰 > 리워드 안내 참고
- 콘텐츠 다운로드 또는 바로보기 완료 후 리뷰 작성 시 익일 제공
가장 와 닿는 하나의 키워드를 선택해주세요.
총 5MB 이하로 jpg,jpeg,png 파일만 업로드 가능합니다.
신고 사유를 선택해주세요.
신고 내용은 이용약관 및 정책에 의해 처리됩니다.
허위 신고일 경우, 신고자의 서비스 활동이 제한될 수
있으니 유의하시어 신중하게 신고해주세요.
이 글을 작성한 작성자의 모든 글은 블라인드 처리 됩니다.
구매 후 90일 이내 작성 시, e교환권 100원 적립
eBook 문장수집은 웹에서 직접 타이핑 가능하나, 모바일 앱에서 도서를 열람하여 문장을 드래그하시면 직접 타이핑 하실 필요 없이 보다 편하게 남길 수 있습니다.
차감하실 sam이용권을 선택하세요.
차감하실 sam이용권을 선택하세요.
선물하실 sam이용권을 선택하세요.
-
보유 권수 / 선물할 권수0권 / 1권
-
받는사람 이름받는사람 휴대전화
- 구매한 이용권의 대한 잔여권수를 선물할 수 있습니다.
- 열람권은 1인당 1권씩 선물 가능합니다.
- 선물한 열람권이 ‘미등록’ 상태일 경우에만 ‘열람권 선물내역’화면에서 선물취소 가능합니다.
- 선물한 열람권의 등록유효기간은 14일 입니다.
(상대방이 기한내에 등록하지 않을 경우 소멸됩니다.) - 무제한 이용권일 경우 열람권 선물이 불가합니다.
첫 구매 시 교보e캐시 지급해 드립니다.
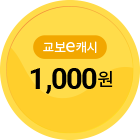
- 첫 구매 후 3일 이내 다운로드 시 익일 자동 지급
- 한 ID당 최초 1회 지급 / sam 이용권 제외
- 구글바이액션을 통해 교보eBook 구매 이력이 없는 회원 대상
- 교보e캐시 1,000원 지급 (유효기간 지급일로부터 7일)