Natural Language Processing and Computational Linguistics
2018년 06월 29일 출간
- eBook 상품 정보
- 파일 정보 PDF (7.60MB)
- ISBN 9781788837033
- 지원기기 교보eBook App, PC e서재, 리더기, 웹뷰어
-
교보eBook App
듣기(TTS) 가능
TTS 란?텍스트를 음성으로 읽어주는 기술입니다.
- 전자책의 편집 상태에 따라 본문의 흐름과 다르게 텍스트를 읽을 수 있습니다.
- 이미지 형태로 제작된 전자책 (예 : ZIP 파일)은 TTS 기능을 지원하지 않습니다.
PDF 필기가능 (Android, iOS)
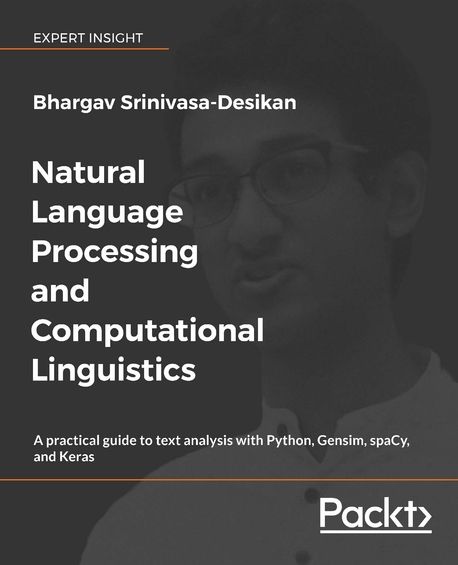
쿠폰적용가 17,100원
10% 할인 | 5%P 적립이 상품은 배송되지 않는 디지털 상품이며,
교보eBook앱이나 웹뷰어에서 바로 이용가능합니다.
카드&결제 혜택
- 5만원 이상 구매 시 추가 2,000P
- 3만원 이상 구매 시, 등급별 2~4% 추가 최대 416P
- 리뷰 작성 시, e교환권 추가 최대 200원
작품소개
이 상품이 속한 분야
1: WHAT IS TEXT ANALYSIS?
2: PYTHON TIPS FOR TEXT ANALYSIS
3: SPACY'S LANGUAGE MODELS
4: GENSIM ? VECTORIZING TEXT AND TRANSFORMATIONS AND N-GRAMS
5: POS-TAGGING AND ITS APPLICATIONS
6: NER-TAGGING AND ITS APPLICATIONS
7: DEPENDENCY PARSING
8: TOPIC MODELS
9: ADVANCED TOPIC MODELING
10: CLUSTERING AND CLASSIFYING TEXT
11: SIMILARITY QUERIES AND SUMMARIZATION
12: WORD2VEC, DOC2VEC, AND GENSIM
13: DEEP LEARNING FOR TEXT
14: KERAS AND SPACY FOR DEEP LEARNING
15: SENTIMENT ANALYSIS AND CHATBOTS
▶What this book covers
? Chapter 1, What is Text Analysis? There is no time like now to do text analysis - we have an abundance of easily available data, powerful and free open source tools to conduct our analysis and research on Machine Learning, Computational Linguistics, and computing with text is progressing at a pace we have not seen before. In this chapter, we will go into details about what exactly text analysis is, and the motivations for studying and understanding text analysis.
? Chapter 2, Python Tips for Text Analysis. We mentioned in Chapter 1, What is Text Analysis, that we will be using Python throughout the book because it is an easy-to-use and powerful language. In this chapter, we will substantiate these claims, while also providing a revision course in basic Python for text analysis. Why is this important? While we expect readers of the book to have a background in Python and high-school math, it is still possible that it’s been a while since you’ve written Python code - and even if you have, Python code you write during text analysis and string manipulation is quite different from, say, building a website using the web framework Django.
? Chapter 3, spaCy’s Language Models. While we introduced text analysis in the previous chapter, we did not discuss any of the technical details behind building a text analysis pipeline. In this chapter, we will introduce you to spaCy’s Language Model - these will serve as the first step in text analysis, and are the first building block in our pipelines. Also, we will introduce the reader to spaCy and how we can use spaCy to help us in our text analysis tasks, as well as talk about some of it’s more powerful functionalities, such as POStagging and NER-tagging. We will finish up with an example of how we can preprocess data quickly and efficiently using spaCy.
? Chapter 4, Gensim ?Vectorizing Text and Transformations and n-grams. While we have worked with raw textual data so far, any Machine Learning or information retrieval related algorithm will not accept data like this - which is why we use mathematical constructs called Vectors to help let the algorithms make sense of the text. We will introduce gensim as the tool to conduct this transformation, as well as scikit-learn, which will be used before we plug in the text to any sort of further analysis. A huge part of preprocessing is carried on over when we start our vectorization - bi-grams, tri-grams, and n-grams, as well using term frequencies to get rid of some words which we deem to not be useful.
? Chapter 5, POS-Tagging and Its Applications. Chapters 1 and 2 introduced text analysis and Python, and chapters 3 and 4 helped us set-up our code for more advanced text analysis. This chapter discusses the first of such advanced techniques - part of speech tagging, popularly called POS-tagging. We will study what parts of speech exist, how to identify them in our documents, and what possible uses these POS-tags have.
? Chapter 6, NER-Tagging and Its Applications. In the previous chapter, we saw how we can use spaCy’s language pipeline - POS-tagging is a very powerful tool, and we will now explore itsanother interesting usage, NER-tagging. We will discuss what exactly this is from a both linguistic and text analysis point of view, as well as detailing examples of its usage, and how to train our own NER-tagger with spaCy.
? Chapter 7, Dependency Parsing. We saw in Chapters 5 and 6 how spaCy’s language pipeline performs a variety of complex Computational Linguistics algorithms, such as POS-tagging and NER-tagging. This isn’t all spaCy packs though, and in this chapter we will explore the power of dependency parsing and how it can be used in a variety of contexts and applications. We will have a look at the theory of dependency parsing before moving on to using it with spaCy, as well as training our own dependency parsers.
? Chapter 8, Topic Models. Until now, we dealt with ...
▶ Preface
Modern text analysis is now very accessible using Python and open source tools, so discover how you can now perform modern text analysis in this era of textual data.
This book shows you how to use natural language processing, and computational linguistics algorithms, to make inferences and gain insights about data you have. These algorithms are based on statistical machine learning and artificial intelligence techniques. The tools to work with these algorithms are available to you right now - with Python, and tools like Gensim and spaCy.
You'll start by learning about data cleaning, and then how to perform computational linguistics from first concepts. You're then ready to explore the more sophisticated areas of statistical NLP and deep learning using Python, using realistic language and text samples. You'll learn to tag, parse, and model text using the best tools. You'll gain hands-on knowledge of the best frameworks to use, and you'll know when to choose a tool like Gensim for topic models, and when to work with Keras for deep learning.
This book balances theory and practical hands-on examples, so you can learn about and conduct your own natural language processing projects and computational linguistics. You'll discover the rich ecosystem of Python tools you have available to conduct NLP - and enter the interesting world of modern text analysis.
작가정보
Bhargav Srinivasa-Desikan is a research engineer working for INRIA in Lille, France. He is a part of the MODAL (Models of Data Analysis and Learning) team, and has a deep interest in modern text analysis. He works on metric learning, predictor aggregation, and data visualization. He is a regular contributor to the Python open source community, and completed Google Summer of Code in 2016 with Gensim where he implemented Dynamic Topic Models. He is a regular speaker at PyCons and PyDatas across Europe and Asia, and conducts tutorials on text analysis using Python.
이 상품의 총서
Klover리뷰 (0)
- - e교환권은 적립일로부터 180일 동안 사용 가능합니다.
- - 리워드는 5,000원 이상 eBook, 오디오북, 동영상에 한해 다운로드 완료 후 리뷰 작성 시 익일 제공됩니다. (2024년 9월 30일부터 적용)
- - 리워드는 한 상품에 최초 1회만 제공됩니다.
- - sam 이용권 구매 상품 / 선물받은 eBook은 리워드 대상에서 제외됩니다.
- 도서나 타인에 대해 근거 없이 비방을 하거나 타인의 명예를 훼손할 수 있는 리뷰
- 도서와 무관한 내용의 리뷰
- 인신공격이나 욕설, 비속어, 혐오 발언이 개재된 리뷰
- 의성어나 의태어 등 내용의 의미가 없는 리뷰
구매 후 리뷰 작성 시, e교환권 100원 적립
문장수집
- 구매 후 90일 이내에 문장 수집 등록 시 e교환권 100원을 적립해 드립니다.
- e교환권은 적립일로부터 180일 동안 사용 가능합니다.
- 리워드는 5,000원 이상 eBook에 한해 다운로드 완료 후 문장수집 등록 시 제공됩니다. (2024년 9월 30일부터 적용)
- 리워드는 한 상품에 최초 1회만 제공됩니다.
- sam 이용권 구매 상품 / 선물받은 eBook / 오디오북·동영상 상품/주문취소/환불 시 리워드 대상에서 제외됩니다.
구매 후 문장수집 작성 시, e교환권 100원 적립
신규가입 혜택 지급이 완료 되었습니다.
바로 사용 가능한 교보e캐시 1,000원 (유효기간 7일)
지금 바로 교보eBook의 다양한 콘텐츠를 이용해 보세요!
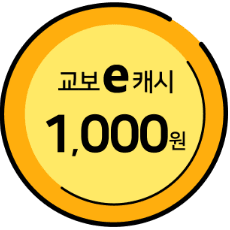
- 구매 후 90일 이내 작성 시, e교환권 100원 (최초1회)
- 리워드 제외 상품 : 마이 > 라이브러리 > Klover리뷰 > 리워드 안내 참고
- 콘텐츠 다운로드 또는 바로보기 완료 후 리뷰 작성 시 익일 제공
가장 와 닿는 하나의 키워드를 선택해주세요.
총 5MB 이하로 jpg,jpeg,png 파일만 업로드 가능합니다.
신고 사유를 선택해주세요.
신고 내용은 이용약관 및 정책에 의해 처리됩니다.
허위 신고일 경우, 신고자의 서비스 활동이 제한될 수
있으니 유의하시어 신중하게 신고해주세요.
이 글을 작성한 작성자의 모든 글은 블라인드 처리 됩니다.
구매 후 90일 이내 작성 시, e교환권 100원 적립
eBook 문장수집은 웹에서 직접 타이핑 가능하나, 모바일 앱에서 도서를 열람하여 문장을 드래그하시면 직접 타이핑 하실 필요 없이 보다 편하게 남길 수 있습니다.
차감하실 sam이용권을 선택하세요.
차감하실 sam이용권을 선택하세요.
선물하실 sam이용권을 선택하세요.
-
보유 권수 / 선물할 권수0권 / 1권
-
받는사람 이름받는사람 휴대전화
- 구매한 이용권의 대한 잔여권수를 선물할 수 있습니다.
- 열람권은 1인당 1권씩 선물 가능합니다.
- 선물한 열람권이 ‘미등록’ 상태일 경우에만 ‘열람권 선물내역’화면에서 선물취소 가능합니다.
- 선물한 열람권의 등록유효기간은 14일 입니다.
(상대방이 기한내에 등록하지 않을 경우 소멸됩니다.) - 무제한 이용권일 경우 열람권 선물이 불가합니다.
첫 구매 시 교보e캐시 지급해 드립니다.
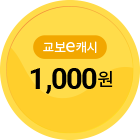
- 첫 구매 후 3일 이내 다운로드 시 익일 자동 지급
- 한 ID당 최초 1회 지급 / sam 이용권 제외
- 구글바이액션을 통해 교보eBook 구매 이력이 없는 회원 대상
- 교보e캐시 1,000원 지급 (유효기간 지급일로부터 7일)